What is AI governance?
AI is more than just a buzzword—it’s a transformative technology that is reshaping industries, driving innovation, and changing the way people work. AI governance is the framework that guides the responsible development, deployment, and use of AI systems to ensure they align with ethical standards and prioritize safety and fairness. As AI gains traction across industries, from healthcare to finance to transportation, the need for effective AI governance is clear.
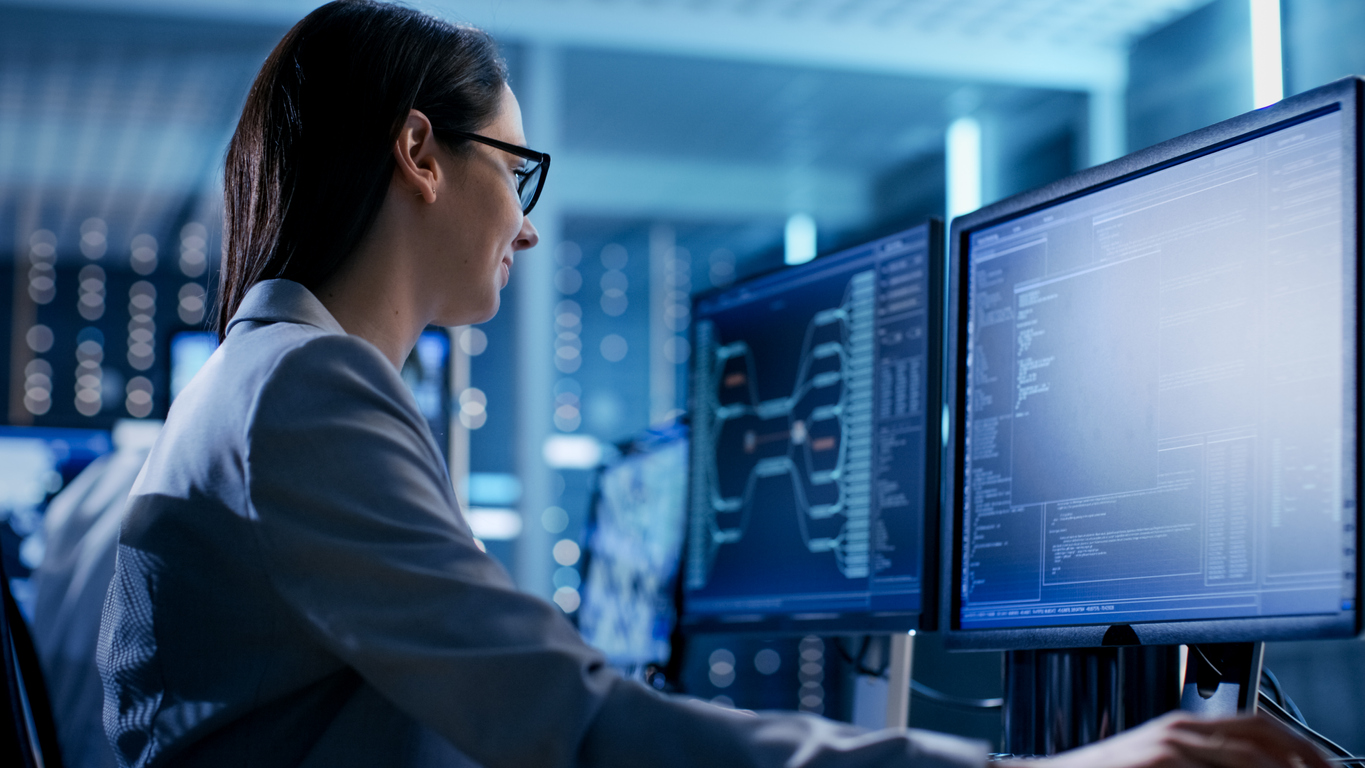
Definition of AI Governance
AI governance aims to balance the opportunities and risks inherent in AI technologies to make sure that they contribute positively to societal progress while upholding fundamental values and ethical principles. It addresses concerns such as data privacy, algorithmic bias, and potential societal impacts of AI applications, such as job displacement.
By establishing clear rules, AI governance aims to promote transparency and accountability in the development and deployment of AI systems. It ensures that stakeholders understand their responsibilities and that decision-making processes are transparent and accessible.
In practice, an AI governance framework encourages collaboration among stakeholders, including policymakers, industry experts, researchers, and the public. A collaborative approach ensures that diverse perspectives and priorities are heard. AI governance safeguards against potential harms while maximizing the benefits of AI technologies for society.
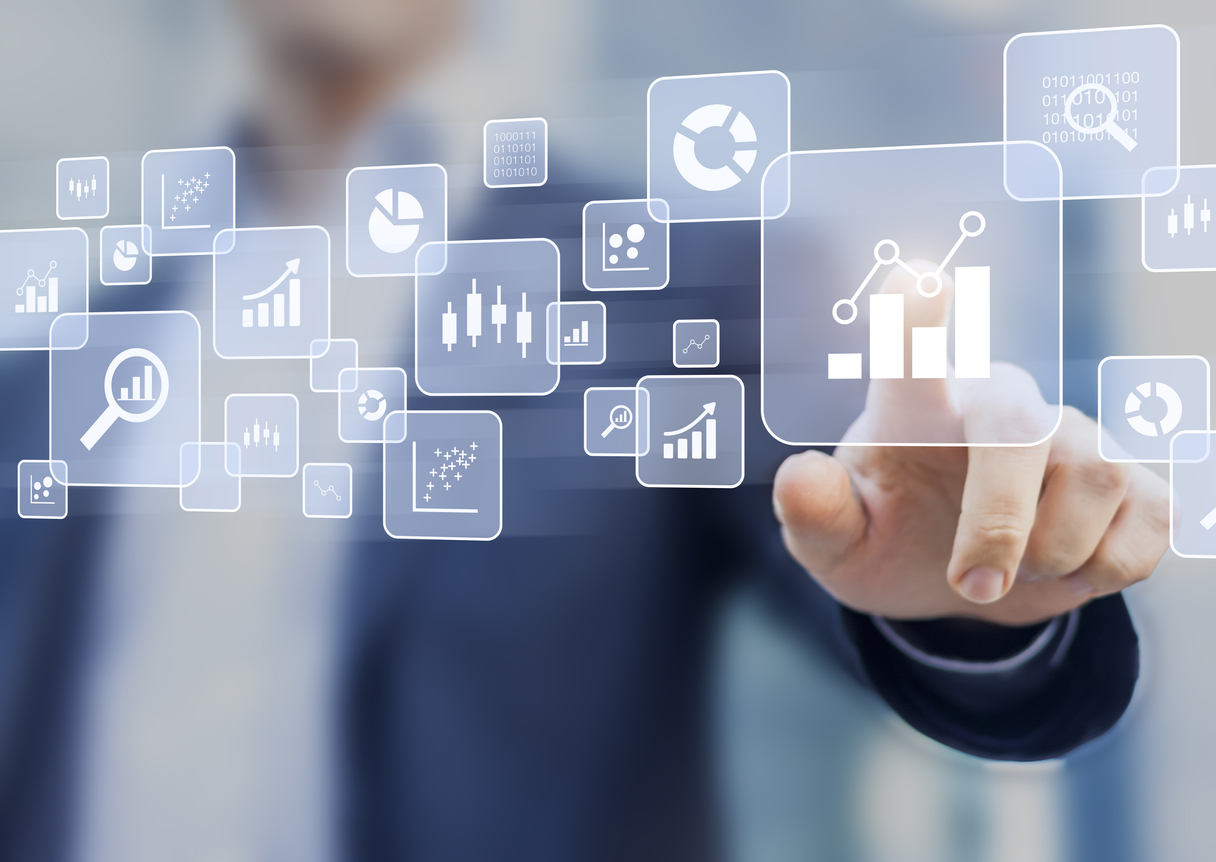
What is AI Governance Like in Action?
Consider the retail industry and its use of AI. In the retail sector, AI is used to enhance customer experiences, optimize operations, and drive sales. Retailers collect vast amounts of customer-specific data, including purchase history, browsing behavior, demographic information, and preferences. Algorithms analyze this data to create tailored marketing campaigns, product recommendations, and promotions for individual customers. By leveraging AI-driven insights, retailers can deliver more relevant and personalized experiences.
AI-powered tools such as chatbots and virtual assistants are being deployed in retail to provide personalized customer assistance. These virtual agents can handle customer inquiries, offer product recommendations, and facilitate transactions, providing round-the-clock support and improving customer engagement. Retailers can even use AI-enabled smart shelves in stores to automatically detect inventory levels and send alerts to store staff, ensuring shelves are always stocked with popular items. AI enables retailers to better understand their customers.
Given the extensive information collected about individual users, data privacy is a key concern. Retailers must adhere to stringent data privacy regulations and take steps to protect customer data from unauthorized access, misuse, or breaches. Retailers should clearly communicate to customers how their data is being collected, stored, and used. This includes providing detailed privacy policies that outline the types of data collected, the purposes for which it is used, and the measures in place to safeguard it. Transparent communication ensures that customers are aware of their rights regarding their personal data.
Another important consideration is data anonymization and encryption. Retailers should anonymize or pseudonymize (that is, de-identify) customer data whenever possible to reduce the risk of individual identification. Sensitive information such as payment card details should be encrypted during both transmission and storage to prevent unauthorized access by malicious actors. Retailers can use strict access controls and authentication mechanisms to limit access to customer data. Role-based access control ensures that employees only have access to the data necessary for their specific roles, reducing the risk of data breaches or misuse. By conducting regular audits, retailers can ensure compliance with data protection regulations, identify areas for improvement, and address any security gaps or weaknesses in their systems. Data privacy in the retail sector requires a thoughtful approach that safeguards customer information.
By establishing clear rules, promoting transparency, and fostering accountability, an AI governance framework can help retail businesses harness the power of AI while protecting the privacy and rights of their customers.
The Necessity for AI Governance
As AI becomes more ubiquitous across more sectors, ethical considerations and adherence to regulatory guidance become increasingly important. The integration of AI tools raises concerns about data privacy, algorithmic bias, and the ethical implications of automated decision-making. Regulatory frameworks such as the General Data Protection Regulation (GDPR) in Europe highlight the need for ensuring that AI technologies are in compliance with data protection laws.
In the absence of robust AI governance, AI systems may introduce risks. These risks include the inappropriate use of data, biased decision-making processes, and breaches of privacy. Without proper oversight, AI algorithms trained on biased data sets may perpetuate or exacerbate existing inequalities, leading to unfair treatment or discrimination against individuals or groups. In addition, inadequate data protection measures can result in unauthorized access to sensitive information, compromising user privacy.
By implementing ethical guidelines and best practices, organizations can mitigate the risks associated with AI technologies. The responsible deployment of AI technologies involves ensuring the protection of user rights, including privacy, autonomy, and non-discrimination.
AI governance goes beyond one-time compliance with regulations and ethical standards. It involves the continuous monitoring and maintenance of ethical standards throughout the development, deployment, and use of AI systems. This includes regular assessments of AI algorithms for biases, vulnerabilities, and unintended consequences. Ongoing training is essential to keep stakeholders informed about evolving ethical considerations.
Transparency and accountability are fundamental pillars of AI governance. Transparency involves making the decision-making processes and algorithms of AI systems accessible to stakeholders, including users, policymakers, and AI developers. This enables them to understand how and why decisions are made. Accountability involves implementing mechanisms to address errors, biases, and unintended consequences.
Main Objectives of AI Governance
AI governance has several key objectives. The overarching goal is to ensure that AI systems operate within legal boundaries and uphold ethical standards while mitigating potential risks and harms. The establishment of guidelines and frameworks for AI systems ultimately is meant to contribute to their safe and beneficial integration into society. At its core, AI governance aims to foster ethical responsibility, regulatory compliance, transparency, accountability, risk management, and stakeholder engagement.
- Ethical Responsibility: Ethical guidelines that govern the development and use of AI systems ensure alignment with societal values and norms. These guidelines address critical issues such as bias, fairness, accountability, transparency, privacy, and the potential societal impacts of AI technologies. By providing a moral compass for developers and users, ethical frameworks guide their decisions and actions in navigating the complexities of AI development and deployment.
- Regulatory Compliance: Governments and regulatory bodies enact laws, regulations, and policies that govern the use of AI technologies. These measures cover data protection, cybersecurity, safety standards, and compliance with existing laws in specific sectors. Their aim is to ensure that AI systems operate within legal boundaries and uphold ethical standards, safeguarding the rights and interests of individuals and society as a whole.
- Transparency and Accountability: Transparency in data usage involves disclosing the sources, types, and quality of data used to train AI models. This includes providing visibility into data collection methods, data processing techniques, and any preprocessing steps. By understanding the data inputs, stakeholders can evaluate the representativeness, diversity, and relevance of the datasets, and accurately assess the robustness and fairness of AI systems. Algorithmic transparency includes providing comprehensive documentation on the underlying algorithms, including their design, functioning, and any updates or modifications. This allows stakeholders to scrutinize the algorithms’ logic, understand their limitations, and assess their potential biases or unintended consequences.
- Risk Management: AI governance involves assessing and managing the risks associated with AI technologies. This includes identifying potential risks such as biases, security vulnerabilities, and societal impacts, and implementing measures to mitigate them. Risk management strategies may involve robust cybersecurity measures, fostering diversity and inclusion in AI development teams, and engaging with stakeholders to understand and address their concerns. Ultimately, effective risk management is essential to ensure the safe, responsible, and ethical use of AI technologies.
- Stakeholder Engagement: AI governance requires collaboration among various stakeholders, including government agencies, industry experts, civil society organizations, academic institutions, ethical review boards, legal experts, international organizations, and end users or consumers. Collaboration among these stakeholders ensures that AI technologies are developed and deployed responsibly, respecting fundamental rights and values. Stakeholders can provide insights and feedback on AI policies and practices, helping to shape a more effective and inclusive governance framework and more sustainable and responsible AI systems.
AI Governance Best Practices
Best practices are determined through a combination of industry standards, regulatory requirements, expert consensus, and evidence gathered from successful implementations. Best practices evolve over time as technologies advance and new challenges emerge.
- Organizations working on AI projects should create formal documents outlining ethical principles and regulatory compliance requirements for data preparation, AI development, and deployment. By setting clear expectations and standards, these policies ensure alignment with organizational values and objectives, promoting ethical and responsible AI practices.
- Designating responsibility for AI governance is crucial for ensuring accountability and consistency across all AI initiatives. By assigning dedicated teams or individuals to oversee AI projects, monitor compliance with policies and regulations, and coordinate with stakeholders, organizations centralize accountability and ensure coherent AI governance approaches.
- Putting compliance mechanisms in place may involve conducting risk assessments, auditing AI systems, and implementing controls to mitigate risks. Organizations need to stay updated on regional, national, and industry-specific regulations and employ proper data management tools and processes to maintain compliance—and minimize legal and reputational risks.
- Training and education programs are crucial for building AI governance capabilities among employees. By providing employees with the knowledge and skills needed to understand and implement AI governance frameworks, organizations empower their workforce to navigate the complexities of AI technologies. Training programs covering cybersecurity, ethical use, and other relevant topics ensure that employees are equipped to uphold ethical standards in AI development and deployment.
- Effective communication with stakeholders is key to fostering transparency, building credibility, and ensuring the success of AI governance initiatives. By engaging with regulators, investors, and customers, organizations can solicit feedback, address concerns, and work toward best practices.
What is Machine Learning Model Governance?
Machine learning model governance supports broader AI governance principles. While AI governance addresses overarching frameworks for overseeing all aspects of AI systems, machine learning model governance focuses specifically on effectively managing the lifecycle of machine learning models. It includes everything from data collection and model training to monitoring, and maintenance. However, both are critical aspects of ensuring the responsible development, deployment, and use of AI technologies.
Key components of machine learning model governance include data governance, model development, model deployment, monitoring and maintenance, and explainability and interpretability.
Data governance involves ensuring the quality, integrity, and privacy of data used for training machine learning models. This includes establishing processes for data collection, storage, processing, and sharing, along with mechanisms for ensuring compliance with data protection regulations and ethical guidelines.
Model development involves following best practices for model development, including data preprocessing, feature selection, and model selection. Thorough testing and validation ensure the accuracy, reliability, and fairness of machine learning models.
Model deployment is when machine learning models are deployed in production environments to ensure security, scalability, and performance. This includes selecting appropriate deployment architectures, integrating models with existing systems, and implementing mechanisms for version control and rollback.
Monitoring and maintenance involve continuously monitoring machine learning models in production to detect performance degradation, data drift, and other issues. Maintenance activities may include updating models with new data, retraining models with improved algorithms, and retiring outdated models.
Explainability and interpretability ensure that machine learning models are transparent and interpretable, allowing stakeholders to understand how decisions are made and why. Explainability is essential for building trust in AI systems and identifying and addressing biases and errors.
By implementing robust machine learning model governance practices, organizations can ensure the responsible development, deployment, and use of machine learning models, ultimately fostering trust, transparency, and accountability in AI technologies.
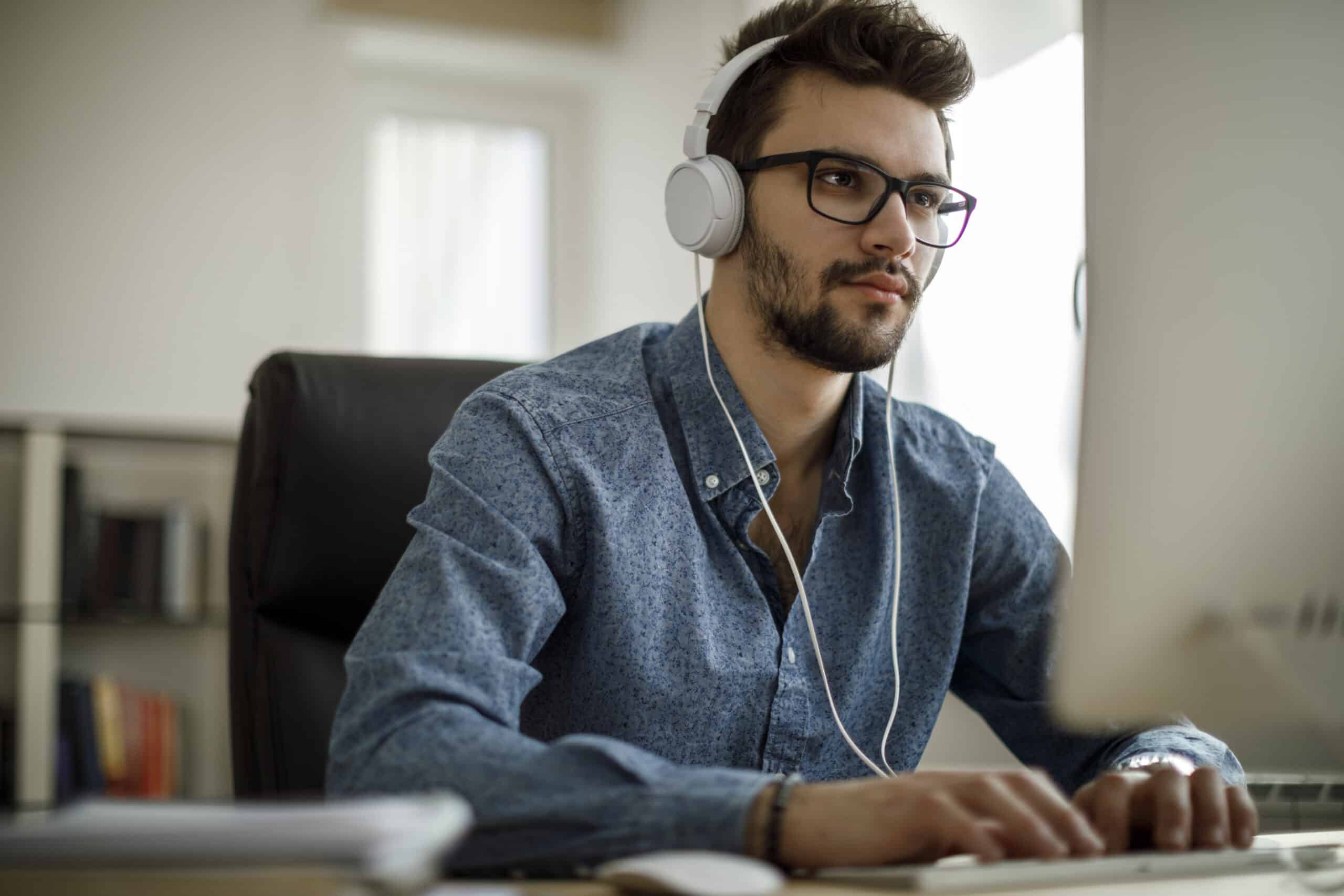
Learn how Reltio can help.
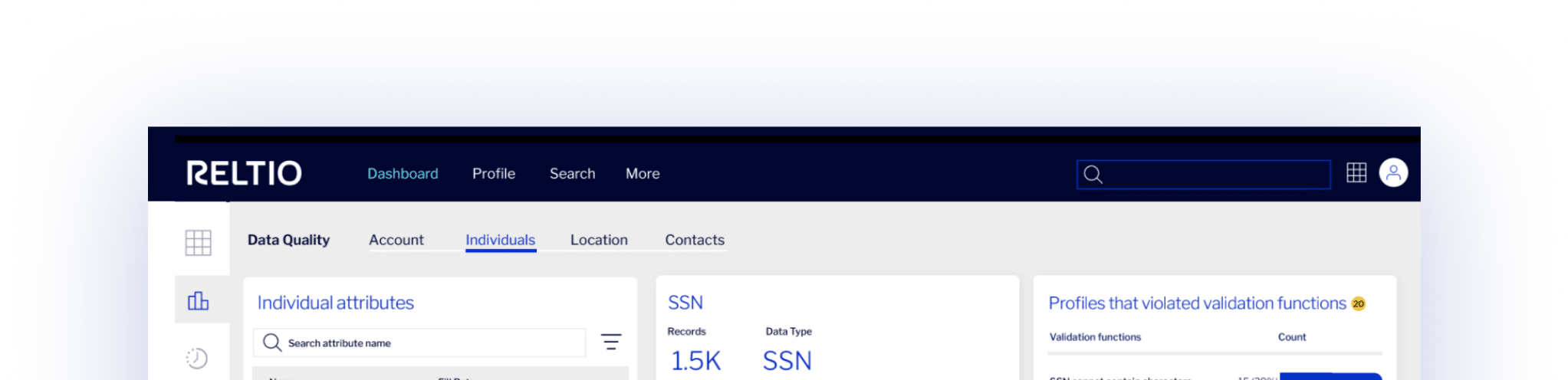