What is Data Governance?
Data governance plays an important role in mitigating the risks that accompany the collecting, storing, and use of data, especially collecting personally identifiable information (PII) at large scale. The power of data governance to manage regulatory compliance, data quality, and data access comes from an established policy-driven approach.
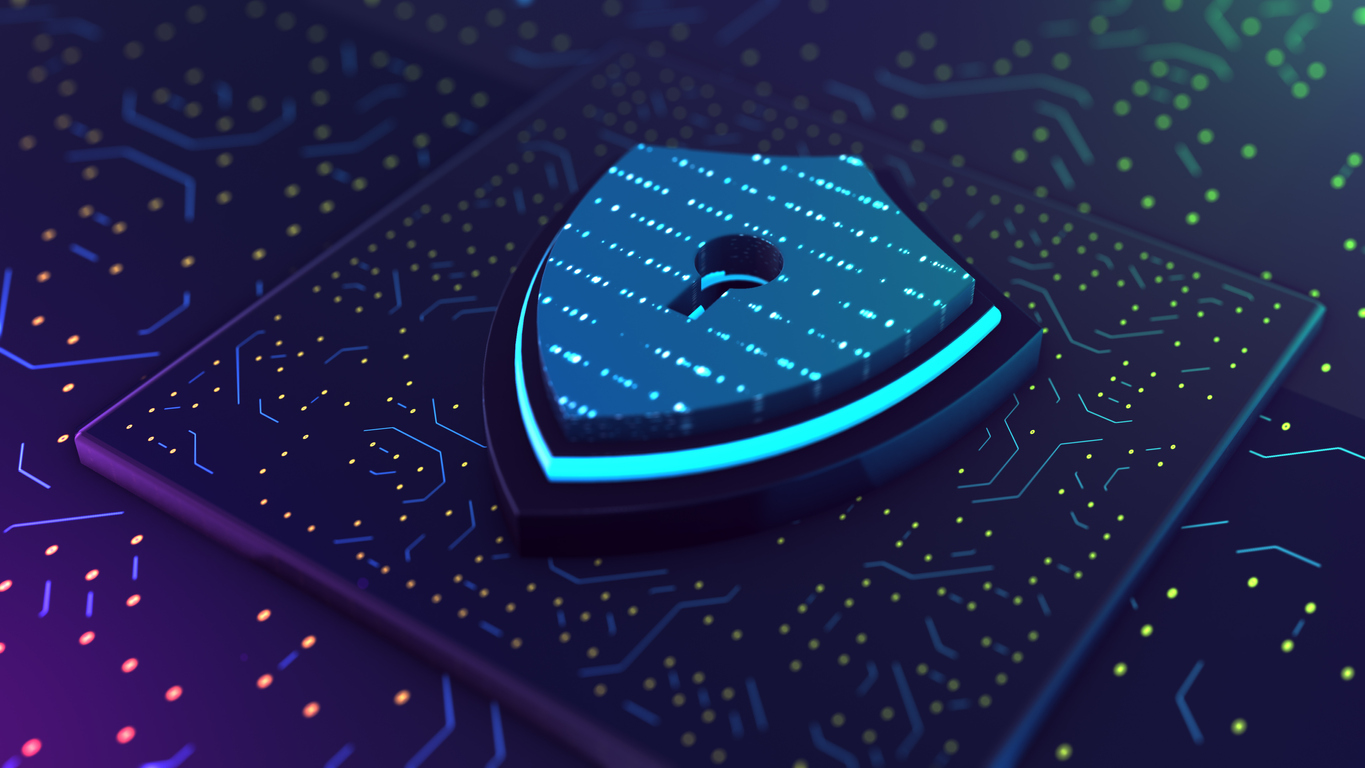
Understanding Data Governance
In general, data governance is a set of rules applied to incoming and stored data where the rules determine what to do to ensure data adheres to regulatory and quality standards. The rules are the governance portion while information technology is used to enforce those rules. The policies, however, are simply the output of a larger process of data governance that should originate from leadership because how data is treated and used impacts more than just the IT department. For this reason, leadership should collaborate with data stakeholders and assign a data steward to lend oversight.
Companies need data governance because the technicalities of managing regulated data are wildly challenged by the three V’s: the volume of data being stored grows exponentially, the varieties of data is increasing, and the ingestion velocity of data is accelerating. When multiplied by the growing number of companies collecting data, the three V’s lead to a glut of data under management, impossible to regulate without automated systems.
Fortunately, intelligent technology is available to enforce data governance policies. Data governance software can enforce policies to restrict access of personal information and other critical data.
Data Governance vs. Master Data Management
Data governance and master data management are separate disciplines that operate within the same domain, so there is often some overlap. Master data is a data set that describes the ‘who’, ‘what’, and ‘where’ in business transactions. A unique ID then can be created and tracked across all systems and transactions for each who, what, and where. A who could be a customer, or employee, a what could be a product, and a where could be a store location. Master data management then is the set of processes executed to maintain that master data, and data governance is the process by which rules and policies are arrived at and maintained.
For further understanding: Master Data Governance: Comprehensive Guide | Reltio
Data Stewardship
Another important concept within data governance is the role of Data Stewards.
According to the Data Governance Institute (DGI): “Data Stewardship is concerned with taking care of data assets that do not belong to the stewards themselves. Data Stewards represent the concerns of others. Some may represent the needs of the entire organization. Others may be tasked with representing a smaller constituency: a business unit, department, or even a set of data themselves.”
DGI’s definition emphasizes the importance of data ownership and the perspective that companies are responsible for protecting data they possess even though it is notionally someone else. The data steward is a formalized position within an organization that acts like an advocate, trustee, and protector of data. This protection encompasses ensuring data quality as well as maximizing data fit and use.
Benefits of Data Governance
The overarching benefit of data governance is to enforce meaning onto data by applying rules to it. Meaning determines the ability of a system to ensure privacy, quality, and fit-for-purpose of its data. A common usage is to protect consumers’ personal information by conforming to regulation. Companies achieve this ultimate goal by adhering best practices, implementing data governance software, and training personnel. With appropriate people, processes, and technology in place, companies can expect the following improvements in their business.
- Greater control to manage risk: Proactively decrease risk by governing data automatically, and specifically with granular controls over how data is processed.
- Make better, more timely decisions: Good data governance would clearly demark the right access to the right data for the right people, ensuring fast and accurate decision making at all levels.
- Improved cost controls: Data helps managers operate more effectively and efficiently.
- Improved access to relevant data: A solid data governance allows companies to confidently democratize their data, sharing access to more people but with proper limits that appropriately protect data.
- Enhanced regulatory compliance: The regulatory climate is complex and poses a substantial cost and risk to businesses that must comply. They must establish robust data governance in their companies to avoid these risks now and in the future.
- Build brand trust and loyalty: Establishing a standard of compliance using data policies helps customers and partners trust that you have their data well protected.
This article, 3 Good Reasons Why Data Governance is the New Cool, presents counterpoints to the argument that data governance is “dull” and lacks value. The three reasons it lays out are:
- Data governance helps you do the cool stuff: Enterprise data strategy and governance approach incorporates AI and ML which helps companies do the “cool stuff” like finding deep actionable insights impossible in siloed data drenched organizations.
- Data governance can deliver the big bucks: Digital transformation doesn’t bring about change without quality data governance. But combined, firms have implemented data governance that have saved millions in costs while opening new opportunities worth even more.
- Data governance has some really amazing pals: Cloud technology has resolved the difficult issue of scalability for many, still teams are challenged to express the value of master data management and data governance. Fortunately, cloud platforms provide specific categories of tools to help them understand their value more.
The Pillars of Data Governance
When speaking of data governance, the term data governance framework crops up. In this respect, a company’s data governance refers to their management of data governance, while a data governance framework is essentially a document. Unique to each organization based on their goals, needs, and features, a data governance framework describes the rules, responsibilities, and processes used to structure their data governance program. It should be referenced by many different actors. It’s meant to provide standardization across the entire organization, covering data standards, data privacy, business strategy, and the responsibilities of key individuals.
A data governance framework begins with a data governance strategy which is the process of coming up with the framework. Along this journey, several data stakeholders, leadership, and end user perspectives are gathered to develop a fitting data scope, organizational structure, data standards and policies, and oversight and metrics.
The need for a process came into being because historically data management was left to IT departments who were not outfitted for the pitfalls of the meaning of data, such as the privacy issues. The onslaught of several data breaches brought to light that leadership and other stakeholders must have input into data governance and therefore data management from the beginning of policy planning. Essentially, because of the potentially sensitive nature of data, its handling has been elevated from just a function of IT.
Because rules and policies play the central part in data governance, a well thought through data governance strategy that specifies appropriate policies is essential. The Data Governance Institute (DGI) defines data governance as “a system of decision rights and accountabilities for information-related processes, executed according to agreed-upon models which describe who can take what actions with what information, and when, under what circumstances, using what methods.”
DGI’s Rules of Engagement helps guide companies when developing their data governance strategy.
- Mission and vision: Define outcome measures, this forms a baseline for collaboration, standardization, and identifying areas of improvement.
- Goals, governance metrics and success measures: Clear measures of success..
- Data rules and definitions: Defined value sets and algorithms.
- Decision rights: Data must be put under the purview of a data steward responsible for collaborating with other data stewards.
- Accountabilities: A data stewards is accountable for their content.
- Controls: Use tools for data entry and data harmony.
- People and organizational bodies: Three general tiers of data users must be classified: those interested in using data, data stakeholders; data governance officers; data stewards.
- Processes (proactive, reactive, and ongoing data governance): Data procedures must be mapped and defined for reference and standardization.
Examples of Data Governance
Data Governance in Finance
Freddie Mac is one of the largest mortgage lenders in the world, collecting and storing a sizable amount of data on borrowers, properties and loans. It is reported that in their single-family line of business alone that Freddie Mac collects 75 data points on each loan, and that they have information for 114 million borrowers and 94 million properties.
It was concluded, in the light of complex legacy mainframe-based infrastructure, increased competition, and inability to nimbly draw insight from their massive databases, that the single-family line of business would need to simplify their architecture. To do so, the transition team set out to devise a practical data governance strategy by first discovering their key areas of improvement.
After consultations and interviews with stakeholders, co-workers, and compliance, audit, and privacy departments, the transformation team compiled a list that identified key areas for improvement. The list contained most of the disciplines under the data management umbrella which would need improvement at Freddie Mac.
The team noted that the consultative process lent valuable credibility to the process which needs buy-in from everyone in the hopes of success. Moreso, the team was sure to continue to publicize the value of good data governance to company stakeholders by creating a brand around it that represented how it will empower them, how it will reduce their hard costs, and how they will gain more control. However, with the transformation process underway, still some hesitation crept in, in which case the head came out to say that the future is coming, the transition will happen so get on board.
Data Governance in Construction
The U.S. Department of Transportation (DOT) noticed an increase in the use of Building Information Modeling (BIM) related technologies in the construction of highways and recognized the data use in BIM presented a potential opportunity for improving lifecycle delivery and management of highway assets. Many other State Departments of Transportations agreed.
In response, the ROADS initiative by Florida Department of Transportation (FDOT) was designed with the goal “to improve data reliability, simplify data sharing across the FDOT, and have readily available and accurate data to make informed decisions.” A task force was assigned to further the data governance needs of the ROADS initiative. After extensive surveys and interviews of over 500 data stakeholders, 63 information gaps were identified. Generally, the consensus was that it is difficult to know what data is available, difficult to access, little to no standardization, no enterprise level view, and a preference for a one-stop-shop.
The data from an Inventory of Information Assets and the Gap Analysis informed the organization of 12 key information enterprise areas. Solutions to address gaps were chosen and planned for. Following that a data governance structure, with four levels, executive team, enterprise data stewards, data stewards, and data custodians, was established to assign roles and responsibilities.
DOTs are large organizations and they recognize that the data governance process is the foundation for better and more accurate decision making. FDOT recognizes this, and is why they have committed to its 5-6 year journey of establishing a reliable full lifecycle data governance model for their highway assets.
https://www.fhwa.dot.gov/construction/bim/pdfs/FHWA_BIM_PPT_FDOT_072920.pdf
Data Governance FAQs
What is data governance?
Data governance is a formalized set of processes, procedures, roles, and responsibilities that provides an overarching structure for decision making and data ownership.
What’s the difference between data governance and data management?
Data governance concerns organization and the process they will take that provides oversight of data under management. Data management encompasses data governance, and concerns itself with the entirety of data to be managed. Sometimes data management is thought about as the tools and practices that support the implementation of a data governance framework.
What is a data owner?
Data owners are senior level staff that are accountable for assigned data sets. They are afforded the budget and resources to define, clean, and maintain their owned data. However, data owners usually pass on to a steward the day-to-day responsibility of managing the data.
What is a data steward?
Data stewards are responsible for managing the day-to-day of a particular data set or more. They report to a data owner.
What is a business glossary?
The business glossary defines the format and use of all critical data elements to a company. This aim is to standardize definitions across the company, contextualize elements, improve understanding, and save time on finding necessary reports. A business glossary is an essential part of data governance.
What is a data dictionary?
Data dictionaries are tables or sets of tables used as repositories for metadata. Mostly these are used in IT contexts.
Ready to see it in action?
Get a personalized demo tailored to your
specific interests.
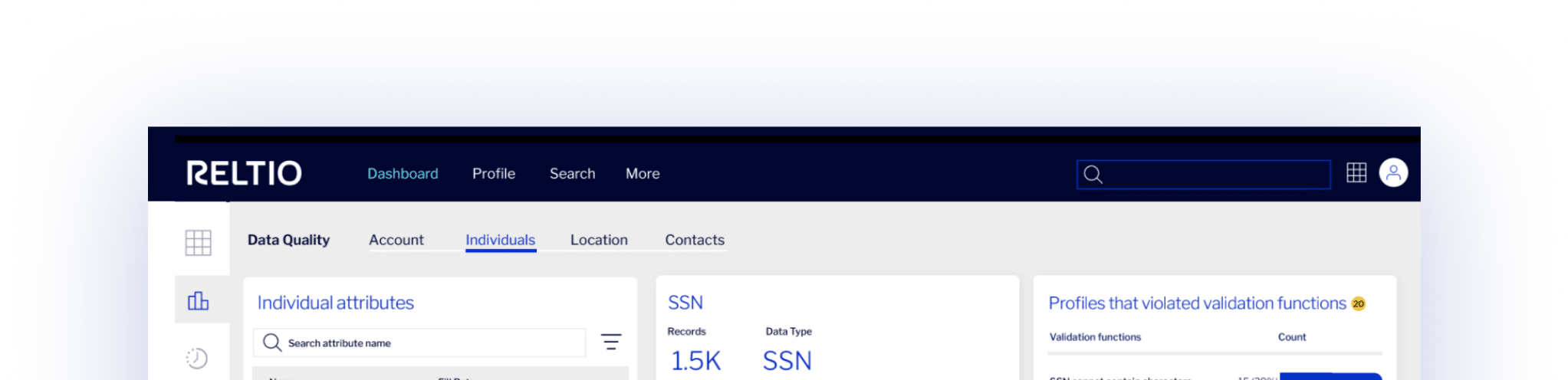