What is Augmented Data Management?
Augmented data management (ADM) is an advanced approach to handling and processing data that combines artificial intelligence (AI), machine learning (ML), and natural language processing (NLP) technologies. It transforms traditional data management by turning manual, time-consuming tasks such as data integration, data cleansing, and data governance into efficient, automated processes.
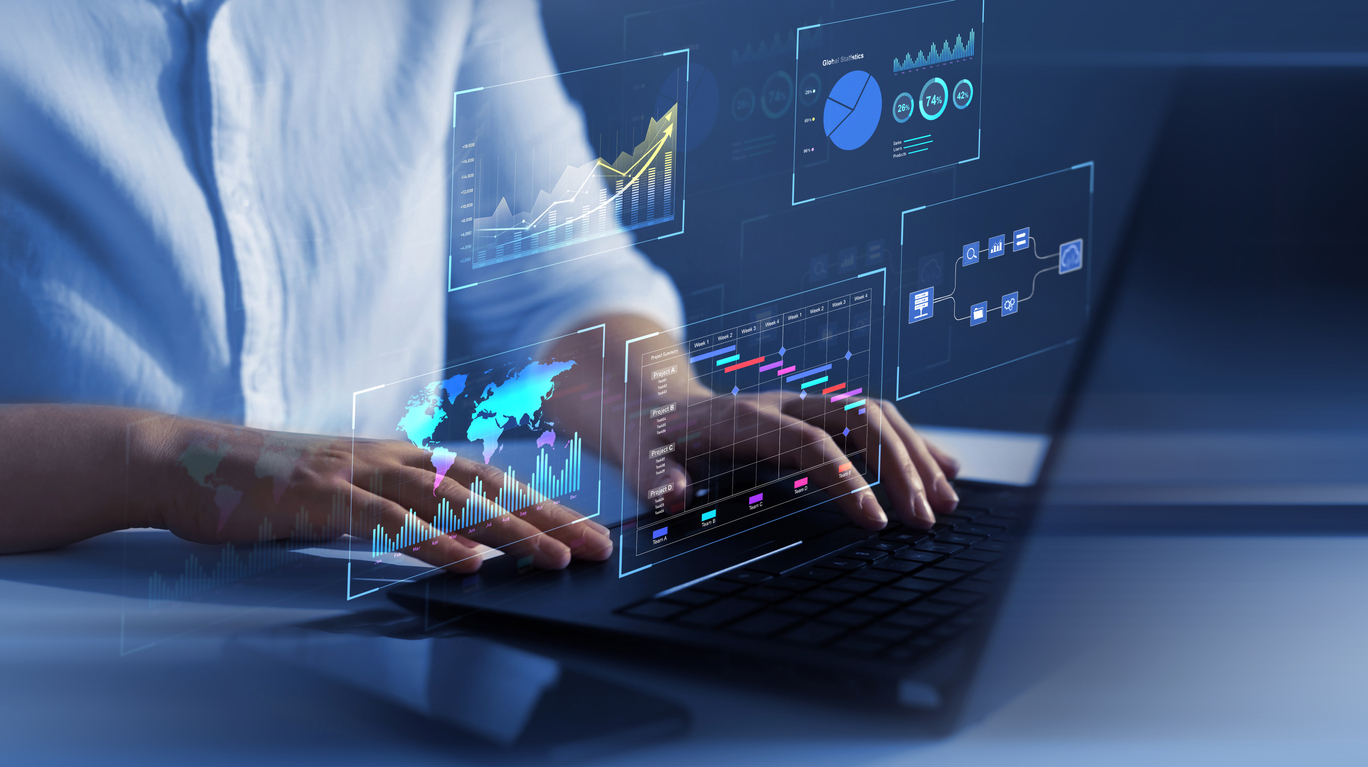
Understanding Augmented Data Management
Augmented data management significantly boosts efficiency in data handling and improves usability. It continuously monitors data quality, automatically detects and corrects anomalies, and helps ensure regulatory compliance. The integration of NLP allows users to interact with data systems through natural language queries, improving data access for even non-technical users.
AI and ML systems provide advanced insights that enable organizations to anticipate trends, identify anomalies, and make data-driven decisions with greater confidence. These predictive analytics capabilities enhance the efficiency and accuracy of data management, giving users better accessibility and actionable insights, leading to better business outcomes.
ADM includes several specific approaches that improve data management transparency and efficiency. These methods aim to make complex data operations more efficient and accessible, helping users interpret, understand, and trust the data management processes and outcomes:
- Automated data quality checks and data profiling
- AI-driven data integration and cleansing
- Intelligent metadata management
- Machine learning-based anomaly detection
- Natural language interfaces for data querying
- Predictive analytics for data governance
- Automated master data management
Augmented data management tools help organizations handle increasing data volume, variety, and velocity while ensuring data quality, compliance, and usability. By automating many aspects of data management, ADM allows organizations to focus on extracting value from their data rather than getting bogged down in manual data handling tasks.
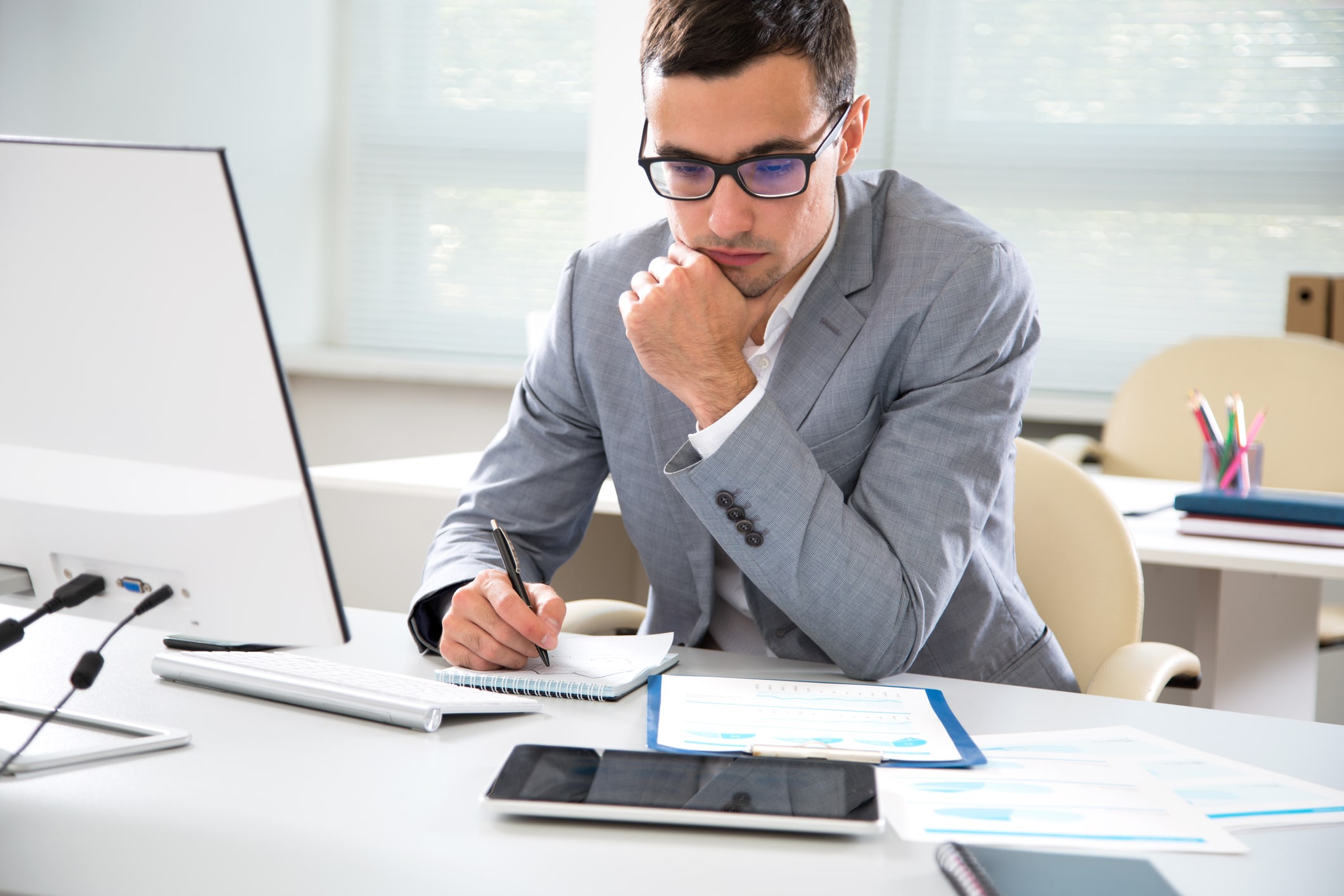
How Augmented Data Management Differs from Traditional Data Management
Augmented data management and traditional data management fundamentally differ in their approaches to handling data. One of the primary differences lies in the level of automation. Traditional data management often involves manual data entry, cleansing, and integration processes. Augmented data management automates these tasks, significantly reducing human intervention and minimizing errors. This automation extends to data quality management as well. While traditional systems often rely on periodic, manual data quality checks, augmented data management continuously monitors data quality in real time, detecting and correcting issues automatically.
Another key differentiator is intelligence. Traditional data management systems typically follow predefined rules and workflows, while ADM incorporates AI and ML to make decisions, adapt to changing data patterns, and provide actionable insights. Intelligence also contributes to augmented data management’s scalability. Traditional systems can struggle with large volumes of data or rapid data growth, but ADM is designed to scale seamlessly as data volumes increase. This is a key benefit in a time when organizations produce more and more data.
Some technical expertise is needed to navigate the interfaces of traditional systems, which are often complex, but augmented data management uses natural language processing. This improves usability, allowing users to interact with data using everyday language. It is a significant step forward in making data more accessible to non-technical users across an organization.
Traditional systems primarily focus on data storage and retrieval. Augmented data management goes further, offering advanced analytics and predictive capabilities that enable companies to not just manage their data, but act on it. This turns data into a crucial advantage.
Traditional systems are often rigid and can be slow to adapt to changing business needs. Augmented data management tools, however, can learn and adapt to new data patterns and business requirements more quickly. This adaptability and flexibility let organizations stay agile when market conditions and business needs change.
Finally, data governance is handled differently in augmented data management systems compared to traditional systems. While traditional systems rely on manual enforcement of data governance policies, ADM can automatically apply these policies and monitor compliance on an ongoing basis. This automated approach to governance helps ensure consistency and reduces the risk of human error in applying data policies.
Augmented data management tools provide a more efficient, accurate, and adaptable approach to handling complex data environments. These advanced capabilities enable organizations to make better use of their data assets and respond more quickly to changing business needs.
Core Components of Augmented Data Management
Augmented data management consists of several core components that work together to transform data management processes. These components make up the foundation of ADM’s capabilities and the value it brings to organizations.
Artificial intelligence and machine learning technologies automate a number of complex data management tasks. They handle processes such as data cleansing, integration, and analysis, significantly reducing manual effort—and mistakes. AI and ML can identify patterns, anomalies, and trends in data that might be impossible for humans to detect, especially when dealing with large volumes of data.
Natural language processing is another key component. It enables users to query and analyze data without needing to understand complex query languages. NLP can interpret user queries, translate them into database queries, and present results in an easily understandable format. It makes data more accessible to everyone in an organization.
Data integration facilitates the seamless combination of data from various sources. ADM systems can bring together data from multiple platforms, ensuring a comprehensive and unified view of an organization’s data. This is crucial for accurate analysis and reporting and eliminates the need for time-consuming manual data consolidation.
Automated data quality management is another core feature of ADM systems. These systems continuously monitor data for quality issues, automatically identifying and correcting errors in real time. This ensures that data remains accurate, consistent, and reliable. Automated data quality management includes tasks such as data validation, standardization, and deduplication, maintaining high data quality without constant manual intervention.
Intelligent metadata management automates the creation and management of metadata, providing context and improving the discoverability of data assets. It makes for better data governance and data utilization. Intelligent metadata management includes features such as automated tagging, data lineage tracking, and impact analysis.
Augmented master data management focuses on creating a comprehensive master data record. ADM enhances this process by automatically consolidating and reconciling data from various sources, creating a single source of truth that can include customer data, product data, supplier data, and more. This ensures consistency across an organization.
Data governance and compliance features round out the core components of ADM systems. These automatically enforce data governance policies and adhere to regulatory standards, reducing risk and enhancing data security. Automated policy enforcement, access control, and audit trail generation help organizations maintain data integrity and meet regulatory requirements.
These core components work together to create a powerful system that can transform an organization’s approach to data management. By automating and enhancing these aspects of data management, ADM enables organizations to focus on getting real value from their data rather than getting bogged down in manual data handling.
The Importance of Augmented Data Management
Organizations are generating and collecting data at unprecedented rates. This volume, variety, and velocity has outpaced traditional data management methods, which rely heavily on manual processes and human intervention. Traditional data management approaches are increasingly inadequate for handling the complexities and scale of modern data environments. Augmented data management addresses these challenges by using advanced technologies to automate and improve data management tasks.
- Augmented data management tools ensure that organizations can continue to derive value from their data without being overwhelmed by it. Traditional data management systems, on the other hand, often struggle to scale effectively as data volumes grow. By automating routine data management tasks, augmented data management frees up time and resources for more strategic activities.
- ADM also improves data quality. Augmented data management tools can continuously monitor data for errors and inconsistencies and automatically correct them. Real-time error detection and correction ensures that data remains accurate, reliable, and ready for analysis. AI and ML algorithms can enforce data quality standards and consistency across different datasets, reducing the risk of data silos and fragmented information.
- Augmented data management enhances data integration by facilitating the seamless combination of data from multiple sources. This provides a unified view of enterprise data, crucial for comprehensive analysis and reporting. It eliminates the need for time-consuming manual data consolidation and ensures that data can be easily shared and used across different systems and applications, boosting its overall value and usability.
- Data governance and compliance are strengthened with ADM. Augmented data management systems can automatically enforce data governance policies, ensuring that data usage complies with internal guidelines and external regulations. This reduces the risk of non-compliance and any associated penalties. Enhanced metadata management and automated documentation provide a clear audit trail of data usage, supporting transparency and accountability.
- AI-driven interfaces and natural language processing capabilities allow even non-technical users to easily access, query, and analyze data. This fosters a more data-driven culture across an organization. Natural language queries and AI-powered recommendations make data access and analysis more intuitive and user-friendly.
- The scalability and flexibility of augmented data management tools allow them to manage large volumes of data from diverse sources without compromising performance. ADM systems can adapt to evolving data environments and business needs, providing long-term value and effectiveness.
- AI and machine learning offer sophisticated analytics capabilities, providing more precise and actionable insights and enhancing decision-making. With real-time data processing, organizations can make decisions based on the most current and relevant information available. The ability to quickly adapt to new data inputs and changing conditions allows organizations to respond to emerging opportunities and challenges.
- Finally, augmented data management provides a competitive advantage. By improving data quality, accessibility, and analytics capabilities, it enables organizations to fully leverage their data assets for innovation. Access to accurate and timely data supports strategic planning and execution, helping organizations stay ahead of competitors and market trends.
The Future of Augmented Data Management
As data continues to grow in importance—and volume—augmented data management is poised to play an increasingly crucial role for organizations. No matter what trends unfold, augmented data management will continue to transform how organizations work with their data. Effectively harnessing augmented data management will position organizations to thrive in an increasingly data-driven world. Several trends are likely to shape the future of ADM:
- Increased AI and ML integration will lead to more sophisticated augmented data management systems. These advanced algorithms will provide more accurate predictions, better anomaly detection, and more nuanced data insights. It could also include explainable AI systems that provide the reasoning behind their predictions in terms humans can easily understand. As AI-driven decisions become more common, ensuring transparency and building trust in automated data management processes will be crucial. This is particularly important in regulated industries or situations where AI decisions need to be audited or explained to stakeholders.
- As IoT devices become more prevalent, augmented data management could adapt to handle data processing and management at the edge—that is, closer to the source of data generation, rather than in a centralized data center or cloud. This would reduce latency, save bandwidth, and enable faster responses in situations where immediate action is needed.
- As NLP technology improves, so could intuitive interfaces for data interaction. Future systems might engage in complex, multi-turn dialogues about data, understanding context and nuance in ways current systems cannot.
- Adaptive learning capabilities could help augmented data management systems become more advanced. These capabilities would enable them to continually improve their performance based on new data and user interactions, potentially customizing their behavior for different users or departments within an organization.
Putting Augmented Data Management into Practice
Augmented data management principles are being integrated into broader data management in numerous ways, including cloud-based solutions. At Reltio, we incorporate ADM concepts into our Connected Data Platform, a cloud-native solution for data unification and multidomain master data management (MDM).
Augmented data management uses AI and machine learning to enhance data management processes. In Reltio’s case, this includes advanced AI technology that accurately identifies and links customer data across different systems. This means:
- You get a single, accurate view of each customer, even when their information is spread across multiple databases or departments.
- The system uses advanced language processing to understand and match customer records, even when there are slight differences in how the information is recorded.
- This process is secure and respects privacy—it uses Reltio’s own protected AI models rather than sending your data to external services.
By automating and improving the data integration and quality management processes, this AI-driven approach makes data management tasks faster and more accurate.
The impact: Better customer insights, improved service, and more targeted marketing, all while maintaining data privacy and security. By incorporating these and other augmented data management capabilities, Reltio’s platform helps you manage your data more effectively so you can derive greater value from your data assets. Our approach enables you to create a unified, reliable view of your critical data entities, supporting better decision-making.
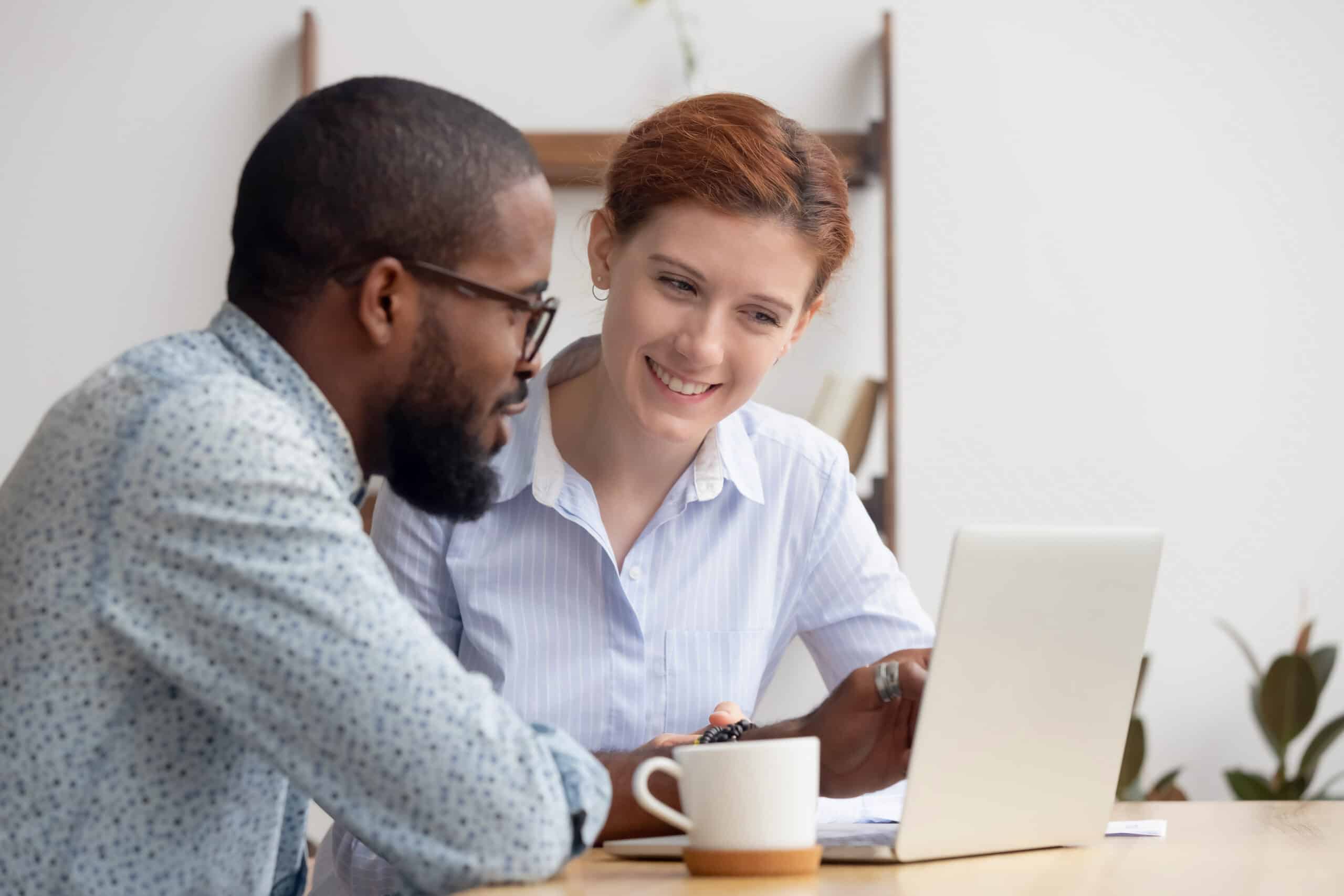
Learn how Reltio can help.
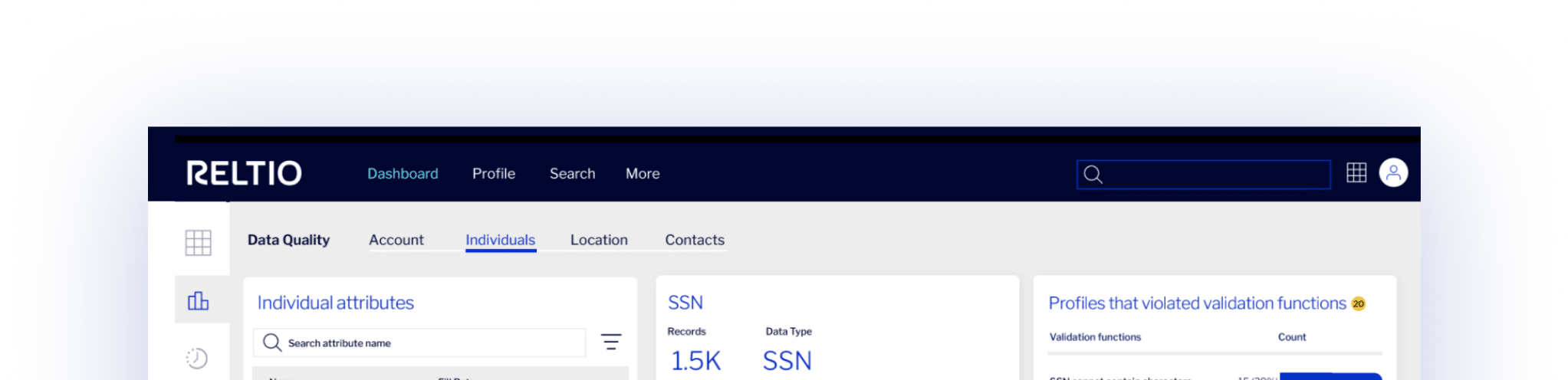