What is a Data Lifecycle?
As businesses increasingly rely on data to drive their operations, the importance of properly managing data throughout its lifecycle becomes critical. Data lifecycle management is a comprehensive approach to managing data from creation to disposal, including how data is collected, stored, processed, and analyzed. With data volume growing exponentially, organizations face numerous challenges in managing their data effectively, such as ensuring data quality, managing storage costs, maintaining data privacy and security, and complying with regulations.
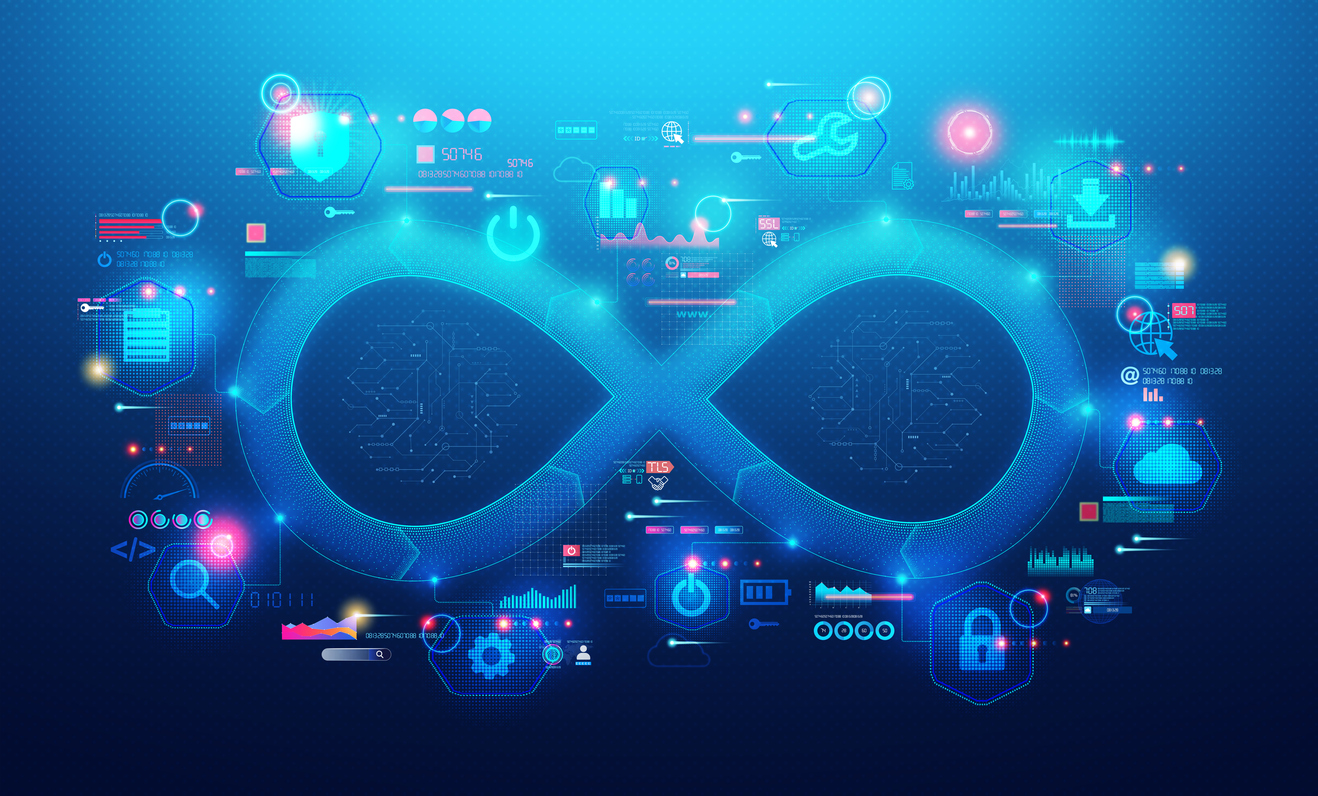
Understanding Data Lifecycles
A data lifecycle refers to the stages that data goes through from its creation or acquisition, through its usage and maintenance, to its eventual disposal. The stages of the data lifecycle may vary depending on the organization, but they generally include the following:
- Data creation or acquisition: Data is created through various means such as capturing user input, sensor data, or scraping from external sources.
- Data storage: Data is stored in a variety of formats such as databases, data warehouses, or data lakes.
- Data processing: Data is transformed, cleansed, aggregated, and analyzed in various ways to derive insights or enable business processes.
- Data sharing: Data is shared with other individuals or systems for various purposes such as collaboration, decision-making, or reporting.
- Data archiving and retention: Data that is no longer actively used may be archived or retained for compliance or legal purposes.
- Data disposal: Data that is no longer needed is securely disposed of or destroyed.
Managing the data lifecycle is important for organizations to ensure that data is accurate, relevant, and available when needed, and that it is properly secured and disposed of when it is no longer needed. A well-defined data lifecycle can also help organizations to optimize their data storage, processing, and sharing practices and ensure compliance with relevant regulations.
Benefits of Data Lifecycle Management
Effective data lifecycle management can help organizations optimize their data usage, reduce storage costs, improve data quality and compliance, and mitigate risks associated with data loss or misuse.
- Reduced storage costs: By automating the data lifecycle process, data that is no longer needed can be identified and deleted, freeing up storage space and reducing storage costs.
- Improved data quality: By managing data throughout its lifecycle, organizations can ensure that data is accurate, consistent, and up-to-date, improving overall data quality.
- Increased compliance: Data lifecycle management can help organizations comply with regulations and standards by ensuring that data is retained for the appropriate amount of time and disposed of properly.
- Better decision-making: By ensuring that data is accurate and up-to-date, organizations can make better-informed decisions based on data-driven insights.
- Improved data security: Data lifecycle management can help organizations identify sensitive data and implement appropriate security measures to protect it.
- Increased efficiency: Automating the data lifecycle process can save time and resources, freeing up staff to focus on more strategic tasks.
Data lifecycle management is an essential component of any organization’s data management strategy, helping to ensure that data is managed effectively throughout its lifecycle to support business goals and objectives.
Data Lifecycle Management vs. Information Lifecycle Management
Data lifecycle management and information lifecycle management are related concepts but they are not the same.
Data lifecycle management (DLM) is the process of managing the entire lifecycle of data, from creation to deletion, while ensuring that it is properly managed and used throughout its life cycle. This includes everything from data acquisition, storage, use, maintenance, retention, and eventual deletion.
Information lifecycle management (ILM) is a broader concept that encompasses data as well as other types of information such as documents, records, and knowledge. ILM is the process of managing the entire lifecycle of information, from creation to deletion or archiving, while ensuring that it is properly managed and used throughout its lifecycle.
While data lifecycle management is focused specifically on managing data, information lifecycle management is a more holistic approach to managing all types of information within an organization.
Best Practices for Data Lifecycle Management
Best practices for data lifecycle management are essential for ensuring the quality and accessibility of data throughout its lifecycle. These practices involve creating clear data management policies, establishing data governance processes, regularly evaluating and optimizing data storage and processing methods, and implementing security measures to protect sensitive data. By following these best practices, organizations can effectively manage their data and derive maximum value from it.
- Define a clear data retention policy: Establish guidelines for how long data should be kept based on legal requirements, business needs, and risk management.
- Use automation to manage data: Use automated processes to help manage the data lifecycle, including data collection, processing, storage, and disposal.
- Secure data at all stages: Implement security controls throughout the data lifecycle to ensure that sensitive data is protected against unauthorized access.
- Implement version control: Establish version control processes for data to ensure that changes are tracked, and older versions of data are retained when necessary.
- Use a centralized data repository: Use a centralized data repository to manage data throughout its lifecycle, including metadata, data lineage, and data quality.
- Perform regular backups and disaster recovery tests: Regular backups and disaster recovery tests should be performed to ensure data availability and prevent data loss.
- Monitor and measure data quality: Monitor data quality throughout the data lifecycle to identify and correct issues and prevent data corruption.
- Establish clear roles and responsibilities: Assign clear roles and responsibilities for managing the data lifecycle, including data stewards and data custodians.
- Incorporate data governance: Ensure that data governance is integrated into the data lifecycle management process to establish policies, procedures, and standards for data management.
- Continuously review and improve the process: Continuously review and improve the data lifecycle management process based on changes in business requirements, technological advancements, and regulatory changes.
Data Lifecycle Frameworks
There are several data lifecycle frameworks that organizations can use to manage their data through its entire lifecycle. Some of the most widely used frameworks include:
- IBM Data Lifecycle Framework: This framework defines the data lifecycle in terms of six stages, including create, store, use, share, archive, and destroy.
- Microsoft Data Lifecycle Framework: This framework defines the data lifecycle in terms of five stages, including collect, enhance, organize, analyze, and govern.
- Informatica Data Lifecycle Management Framework: This framework defines the data lifecycle in terms of six stages, including discovery, profiling, integration, quality, security, and privacy.
- Gartner Data Management Maturity Model: This framework defines the data lifecycle in terms of five stages, including reactive, proactive, managed, optimized, and innovative.
- DAMA International Data Management Body of Knowledge (DAMA-DMBOK): This framework defines the data lifecycle in terms of six stages, including planning, development, implementation, operation, maintenance, and retirement.
Each framework has its own set of guidelines and best practices for managing data throughout its lifecycle. Organizations can choose the framework that best aligns with their needs and goals.
Ready to see it in action?
Get a personalized demo tailored to your
specific interests.
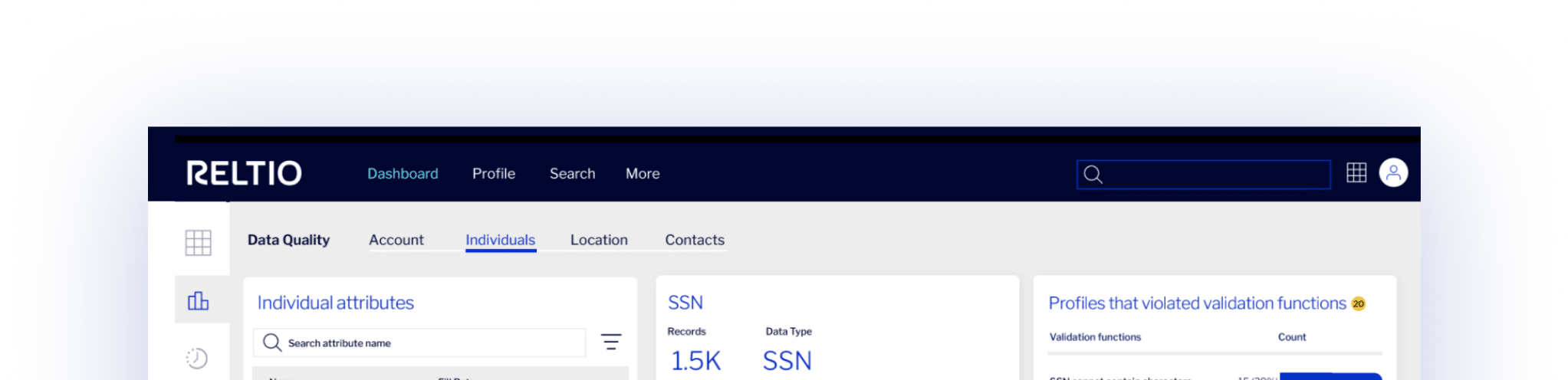