What is Generative AI?
Generative AI is an advanced field of artificial intelligence focused on creating new content by learning patterns and structures from existing data.
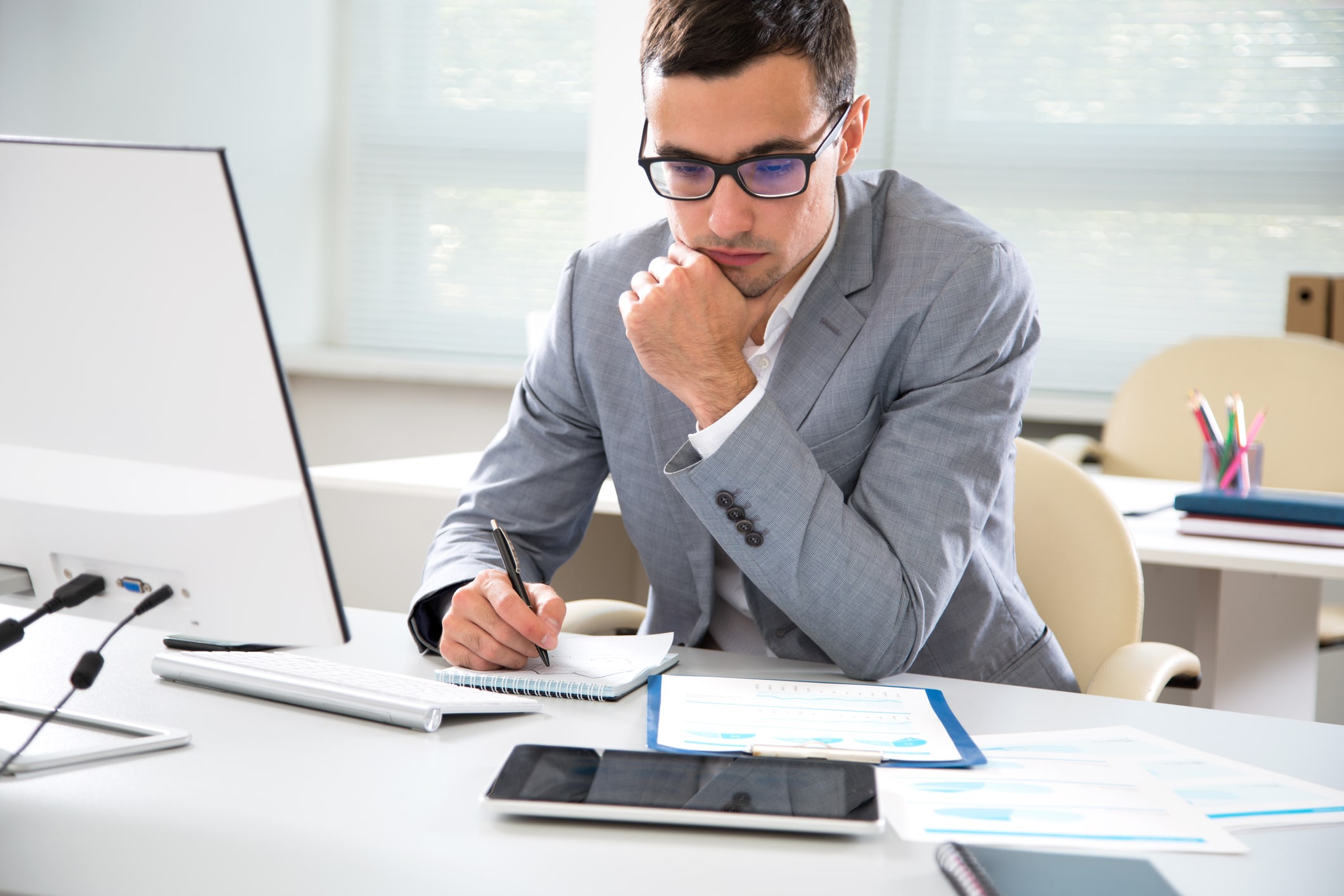
Understanding Generative AI
Unlike traditional AI, which typically analyzes, categorizes, or processes data, generative AI can produce unique content such as text, images, music, and videos. To do this, generative AI leverages sophisticated algorithms and extensive datasets to simulate human creativity.
Generative AI models analyze vast datasets, identifying patterns and structures and applying what they’ve learned to create coherent and contextually relevant outputs. This capability opens up possibilities across various industries, making generative AI a powerful tool for innovation and efficiency. As more organizations turn to generative AI tools for creative and productive tasks, understanding the mechanisms, applications, and best practices of generative AI is increasingly important.
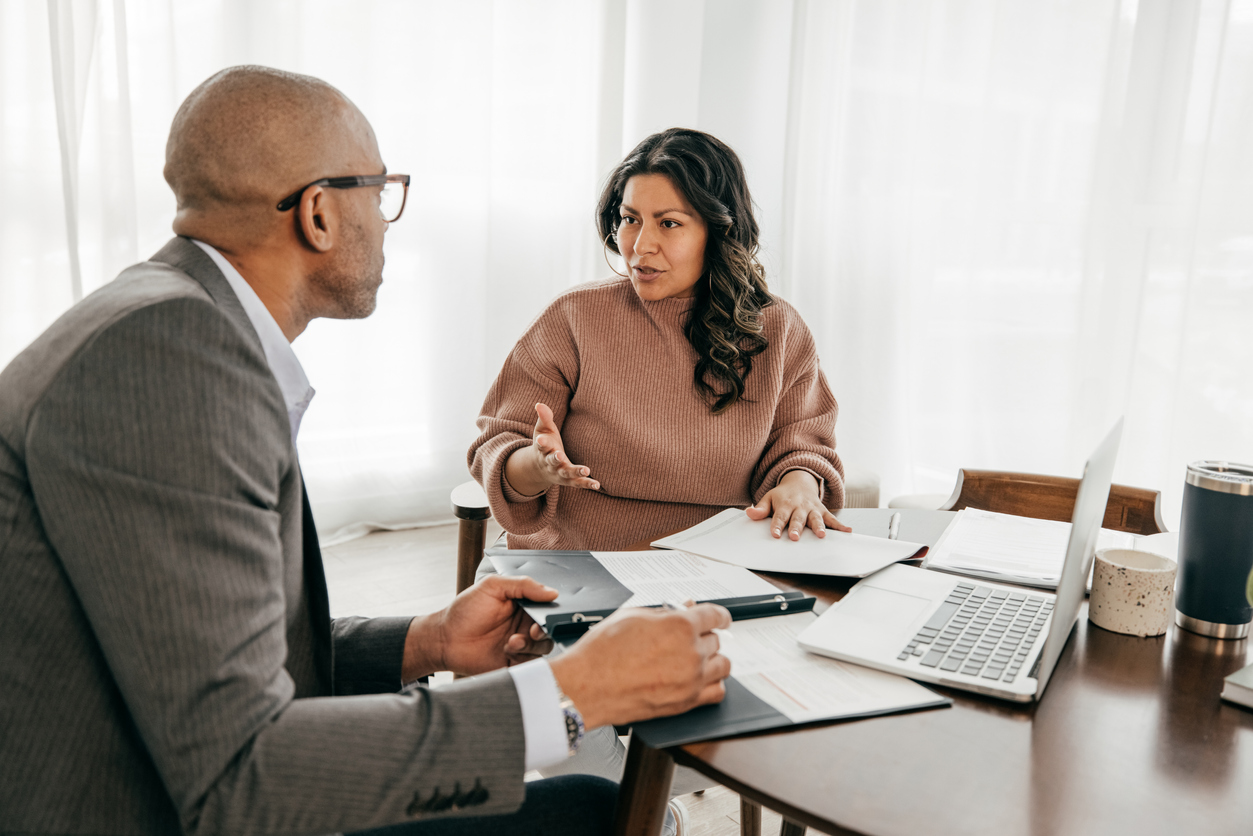
Core Components of Generative AI
Generative AI relies on several key mechanisms and models to create new content. These include neural networks, training data, and specific algorithms designed to generate and refine outputs. Familiarity with these components is key to understanding how generative AI functions and delivers its remarkable results.
Neural networks form the foundation of generative AI systems. These networks are composed of layers of interconnected nodes, or neurons, that process data and learn complex patterns. Designed to mimic the human brain’s neural structure, these networks can identify patterns and structures within existing data to generate new and original content. Neural networks capture complex relationships within the data and enable AI to generate new content that mirrors the patterns it has learned.
The performance and accuracy of generative AI models can vary significantly based on the quality and quantity of the training data. Generative AI models are trained on large datasets that provide diverse examples, allowing the AI to understand and replicate various patterns, structures, and relationships within the data. For example, a generative AI model trained on a large corpus of text learns grammar, context, and style, which it then uses to generate coherent and contextually appropriate text.
Several algorithms are commonly used in generative AI to create new content. Generative Adversarial Networks (GANs) are among the most popular. GANs consist of two neural networks—a generator and a discriminator—that are trained together. The generator creates new content, while the discriminator evaluates its authenticity. Both networks improve over time as the generator produces increasingly realistic content and the discriminator becomes better at spotting fakes.
Another important algorithm is Variational Autoencoders (VAEs). VAEs consist of an encoder and a decoder. The encoder maps input data to a latent space, creating a compressed representation of the data. The decoder then reconstructs the data from this latent representation. By learning the distribution of the data in the latent space, VAEs can generate new data points that are similar to the original dataset. This makes VAEs effective for tasks where it’s crucial to maintain the inherent structure and variability of the data.
Foundational models are large-scale neural networks trained on diverse datasets to understand and generate human-like content across various domains. Models such as OpenAI’s GPT-4 for text and DALL-E for images use deep learning techniques to recognize intricate patterns and relationships within the training data. They often use architectures such as transformers, which enable them to process and generate sequences efficiently. By leveraging extensive pretraining on massive datasets, these models acquire a broad knowledge base, which can be fine-tuned for specific tasks or applications. This approach allows generative AI to produce coherent text, realistic images, and other forms of creative content.
Large Language Models (LLMs) are a specific type of foundational model designed to understand, generate, and manipulate human language with high proficiency. These models are built using deep learning techniques, which excel at handling sequential data. Trained on vast text data from diverse sources, LLMs learn to predict and generate text based on context, capturing nuances in language such as grammar and semantics. Their extensive training enables them to perform a wide range of language tasks, including text completion, translation, summarization, and interacting as conversational agents. LLMs have revolutionized natural language processing (NLP) by providing robust, scalable, and versatile tools for creating human-like text.
How Does Generative AI Work?
Generative AI follows a multi-step process that involves data collection, model training, content generation, and continuous refinement. This process enables AI systems to learn from existing data and create new, original content that mimics human-generated work.
The first step in the generative AI process is data collection and preprocessing. This initial stage involves gathering a large and diverse dataset relevant to the task. For text generation, this might include books, articles, and web content. For image generation, it could consist of labeled images from various categories. The collected data is then cleaned and formatted to ensure consistency and quality. Data cleansing can involve removing duplicates, correcting errors, and normalizing the data. For images, preprocessing might include resizing and augmenting the images to improve model robustness.
The next phase is model training. This involves teaching neural networks to create new content by learning patterns from large datasets. The training phase typically uses architectures such as GANs and VAEs. It requires extensive computational resources and large, diverse datasets to ensure the model accurately captures the underlying structures and can produce realistic and relevant new content.
Once the model is trained, it can engage in content generation. To generate new content, the trained model is given input specifications. For example, a text generation model might receive a prompt or a starting sentence, while an image generation model might receive a textual description or a sample image. The model then uses the patterns and knowledge it has learned during training to produce new content. For text generation, this might involve predicting the next word or sentence based on the given prompt. For image generation, the model creates an image that matches the specified description or style.
The final step in the process is evaluation and refinement. The generated content is evaluated for quality, coherence, and relevance. This evaluation can be performed using automated metrics, human judgment, or a combination of both. Based on the evaluation results, the model may undergo further refinement. That could involve additional training, tweaking the model architecture, or incorporating more diverse training data to address any identified shortcomings.
This iterative process of generation, evaluation, and refinement allows generative AI models to continuously improve their outputs, producing increasingly sophisticated and high-quality content over time.
Generative AI Applications
Generative AI has a wide range of applications across various industries. It has revolutionized content creation, enhancing creative processes and improving efficiency in numerous fields. Generative AI is transforming how people and organizations approach complex tasks and creative endeavors.
Text Generation
When it comes to text generation, generative AI powers chatbots and virtual assistants by enabling them to generate natural and coherent responses to user queries. Models such as OpenAI’s GPT-4 can handle complex conversations, provide customer support, and offer personalized recommendations, improving user experience and operational efficiency. Generative AI can also produce written content such as articles, reports, and social media posts. This allows people to generate high-quality text quickly and efficiently while maintaining a consistent tone and style tailored to specific audiences and purposes.
Additionally, Large Language Models (LLMs) can translate text between languages and summarize long documents, making information more accessible and easier to digest. This capability is valuable in global communication, education, and information dissemination, breaking down language barriers and condensing large volumes of information. For example, LLMs excel in localizing websites, adapting content to different languages and enhancing user engagement and accessibility worldwide.
Image and Video Generation
Image and video generation is another significant area of application for generative AI. These systems can create intricate and novel designs based on textual descriptions or stylistic inputs. Generative AI tools such as DALL-E generate images from text prompts, providing graphic designers and artists with new ways to visualize ideas. This expands creative possibilities and streamlines the design process. In the entertainment industry, generative AI helps produce new visual content for movies, video games, and virtual environments. It can generate realistic landscapes, characters, and special effects, reducing the time and cost associated with traditional animation and visual effects production.
Music and Audio Generation
In music and audio production, AI systems can compose original music by learning patterns from various musical styles and genres. This capability can be used in film scores, video games, advertising, and more. It helps composers explore new creative directions and produces background music at scale. Generative AI can also create sound effects and ambient sounds for multimedia projects, enhancing the auditory experience in virtual environments, games, and films, providing richer and more immersive experiences.
Healthcare
Generative AI is also making significant strides in healthcare applications. It accelerates the drug discovery process by predicting molecular structures and generating potential drug candidates. This can significantly reduce the time and cost associated with developing new medications, leading to faster advancements in medical treatments and therapies. AI models can also generate high-quality medical images, such as MRI or CT scans, which can be used for training purposes or to augment diagnostic processes.
By creating synthetic yet realistic medical images, generative AI helps train healthcare professionals and improves diagnostic accuracy, especially in areas with limited access to medical imaging resources. In addition, generative AI produces synthetic datasets that can be used for research and training machine learning models without compromising patient privacy. This is crucial for developing and testing new medical algorithms while ensuring data diversity and compliance with privacy and security regulations.
Benefits of Generative AI
Generative AI offers numerous benefits across various industries and applications, enhancing creativity, increasing efficiency, and driving innovation. These advantages are transforming how businesses operate and how creative professionals approach their work.
Generative AI is a powerful tool for boosting human creativity by spurring new ideas, designs, and concepts that artists, writers, and designers can build upon. AI-generated art and design provide fresh inspiration, while AI-written text can help overcome writer’s block. This leads to innovative and diverse outputs that might not be achieved by humans alone. Generative AI identifies unique patterns and combinations that humans might overlook, allowing for the creation of entirely new art forms and design paradigms, pushing the boundaries of traditional creative processes. Artists and designers can use AI-generated content as a starting point or integrate it into their work to produce novel and engaging pieces.
Increased efficiency is another significant advantage of generative AI. Generative AI automates and accelerates repetitive and time-consuming tasks, significantly increasing productivity. In content creation, AI can quickly generate large volumes of text, images, or music, freeing up humans to focus on the more strategic and complex aspects of their work. Automation reduces the time and effort required to produce high-quality content. Generative AI also allows for scalable content production, as businesses can use AI to generate personalized marketing materials, product descriptions, and customer communications at scale. This is particularly valuable in industries where large amounts of tailored content are needed to engage customers effectively.
Generative AI is a powerful driver of innovation and discovery. It significantly accelerates research and development processes, particularly in pharmaceuticals and materials science. By generating and testing new molecular structures or materials, AI identifies promising candidates more quickly than is possible with traditional methods. This shortens the time to market for new drugs and innovative materials, fostering rapid advancements in science and technology. Generative AI enables the exploration of new possibilities by generating hypotheses, simulations, and models that can be tested and refined. In scientific research, AI-generated simulations predict outcomes and identify potential solutions to complex problems.
Improved quality and consistency is another key benefit of generative AI. AI-generated text maintains coherent grammar, style, and context, while AI-generated images exhibit detailed and realistic features. This high-quality output ensures that the content meets desired standards and is suitable for professional use. Generative AI also ensures content remains consistent in tone, style, and messaging across various platforms and formats, which can strengthen brand identity and ensure a cohesive user experience.
Generative AI contributes to significant cost reduction for businesses. By automating content creation and other repetitive tasks, it substantially reduces production costs. Businesses save on labor and resources that would otherwise be spent on manual content creation. This is particularly beneficial for small and medium-sized enterprises with limited budgets for content production. Generative AI optimizes the use of available resources, allowing businesses to achieve more with less. AI-generated materials can be produced quickly and efficiently, enabling companies to allocate resources to other strategic initiatives.
Generative AI Best Practices
As generative AI continues to evolve and find new applications, it’s crucial to implement best practices to ensure its responsible and effective use. These best practices help organizations maximize the benefits of generative AI while mitigating potential risks and ethical concerns.
Quality Data Collection
Quality data collection is fundamental to the success of generative AI systems. Organizations must ensure that the data collected for training is comprehensive, diverse, and representative of the intended use case. This involves gathering data from a wide range of sources and carefully vetting its quality and relevance. The collected data should be cleaned and preprocessed to remove errors, duplicates, and irrelevant information. This ensures that the model learns from accurate and relevant examples, leading to more reliable and effective outputs.
Bias Mitigation
Bias mitigation is a critical consideration in generative AI development. Teams must actively work to identify and mitigate biases in the training data. This involves scrutinizing the dataset for imbalances or stereotypes that could influence the model’s outputs. Addressing identified biases and promoting fairness and inclusivity in AI outputs is essential for creating ethical and trustworthy generative AI systems.
Evaluation and Validation
Regular evaluation and validation are necessary to maintain the performance and reliability of generative AI models. Organizations should continuously evaluate their models’ performance using robust validation frameworks. This includes using metrics that align with the intended application. For text generation, metrics could include accuracy, coherence, diversity, and relevance. For image generation, metrics could include realism and fidelity. Regular testing is important because it ensures a model maintains its performance over time. Automated tools can track key performance indicators (KPIs) and detect anomalies or drifts in the model’s behavior, allowing for early identification of any issues with the model’s reliability and effectiveness.
Generative AI models require regular updates and retraining to maintain their relevance and performance. As new data becomes available or as user needs evolve, models should be retrained to incorporate fresh information and adapt to changing conditions. This helps ensure that generative AI systems remain current and effective in their intended applications.
Ethical Considerations
Ethical considerations must be incorporated into the model development process. Clear guidelines can ensure that the AI respects privacy, avoids generating harmful content, and adheres to societal norms and values. Ethical frameworks should be regularly reviewed and updated to reflect evolving societal standards and technological capabilities.
Security Measures
Implementing robust security measures is crucial to protect generative AI systems from unauthorized access, data breaches, and other cyber threats. This includes using encryption, access controls, and regular security audits to safeguard both the model and the data it processes. As generative AI often deals with sensitive or proprietary information, maintaining strong security practices is essential for maintaining trust and complying with data protection regulations.
Regulations
Adherence to regulations is a critical aspect of responsible generative AI deployment. Organizations must ensure that their generative AI systems comply with relevant laws and regulations, such as GDPR, CCPA, or other data protection and privacy laws. Regular reviews and updates should be conducted to ensure alignment with regulatory changes and industry standards.
Transparency and Accountability
Transparency and accountability are fundamental principles in generative AI best practices. Organizations should maintain transparency about the use and capabilities of their generative AI systems. This includes clearly communicating the purpose, limitations, and potential risks of the AI to users and stakeholders. Establishing accountability mechanisms to address any issues that arise ensures builds trust with users and the wider public.
By adhering to these best practices, organizations can harness the power of generative AI while minimizing risks and ensuring ethical, effective, and responsible use of this transformative technology.
Key Requirements for Effective Generative AI
For generative AI to produce high-quality, reliable outputs, it requires a solid foundation of clean, trusted, and well-organized data. By addressing data management fundamentals, organizations can enhance the effectiveness and reliability of their generative AI systems. There are several key factors that contribute to the effectiveness of generative AI systems:
- Data Quality: The accuracy and reliability of generative AI outputs are largely determined by the quality of the training data. Having high-quality data that is free from errors, duplicates, and inconsistencies is critical. Rigorous data cleansing processes can ensure the data used to train generative AI models is accurate and reliable.
- Data Unification: Data unification brings together data from various sources, creating a single, coherent dataset. This unified data provides generative AI models with a complete and accurate representation of the information, leading to better and more contextually relevant outputs.
- Master Data Management (MDM): Effective MDM focuses on ensuring that critical business data is consistent, accurate, and up-to-date across all systems and departments. For generative AI, this means having a reliable single source of truth for key data entities, improving the AI’s ability to generate accurate and consistent content.
- Data Governance: Implementing strong data governance practices means that data used in generative AI systems is managed according to both organizational policies and regulatory requirements. This includes managing data access, ensuring data privacy, and maintaining data integrity.
- Real-Time Data Updates: For generative AI models to stay current and relevant, they need access to the most up-to-date information. Real-time data integration and updates ensure that an AI system can generate content based on the latest available data.
- Contextual Understanding: Generative AI systems benefit from a rich understanding of the relationships among different data points. This contextual information helps the AI generate more nuanced and relevant outputs.
Leveraging Generative AI with Reltio
Reltio’s solutions directly address the key requirements for effective generative AI. Our Connected Data Platform provides the essential infrastructure needed to maximize the potential of generative AI technologies. For example, our Connected Graph technology captures and maintains complex relationships among data entities, helping generative AI produce more contextually aware results. Our robust data governance features help organizations maintain control over their data, ensuring that generative AI applications comply with relevant regulations and organizational policies.
Reltio’s Connected Data Platform helps organizations create a solid foundation for their generative AI initiatives and ensure that AI-generated content is not only engaging but also accurate, consistent, and trustworthy.
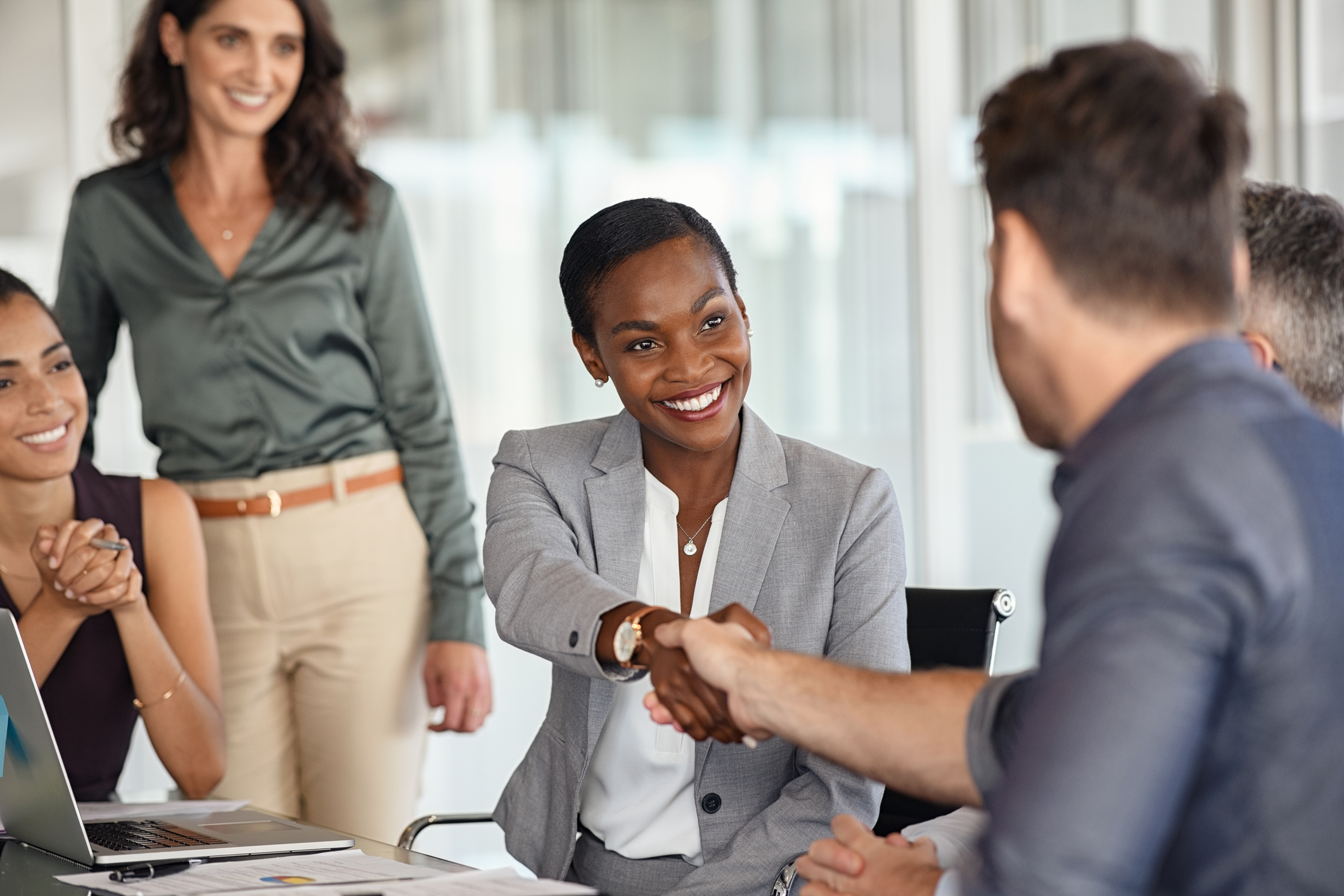
Learn how Reltio can help.
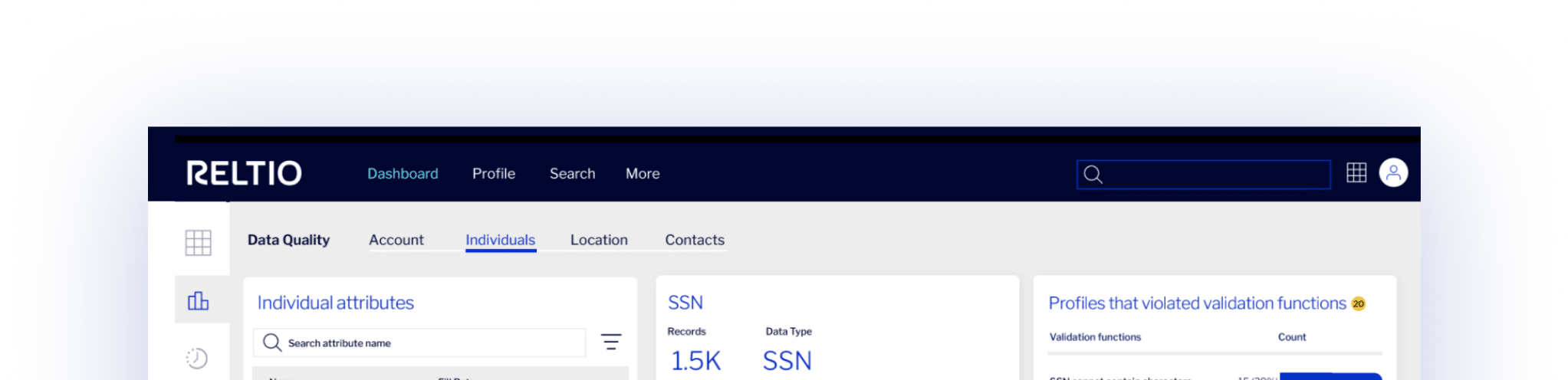