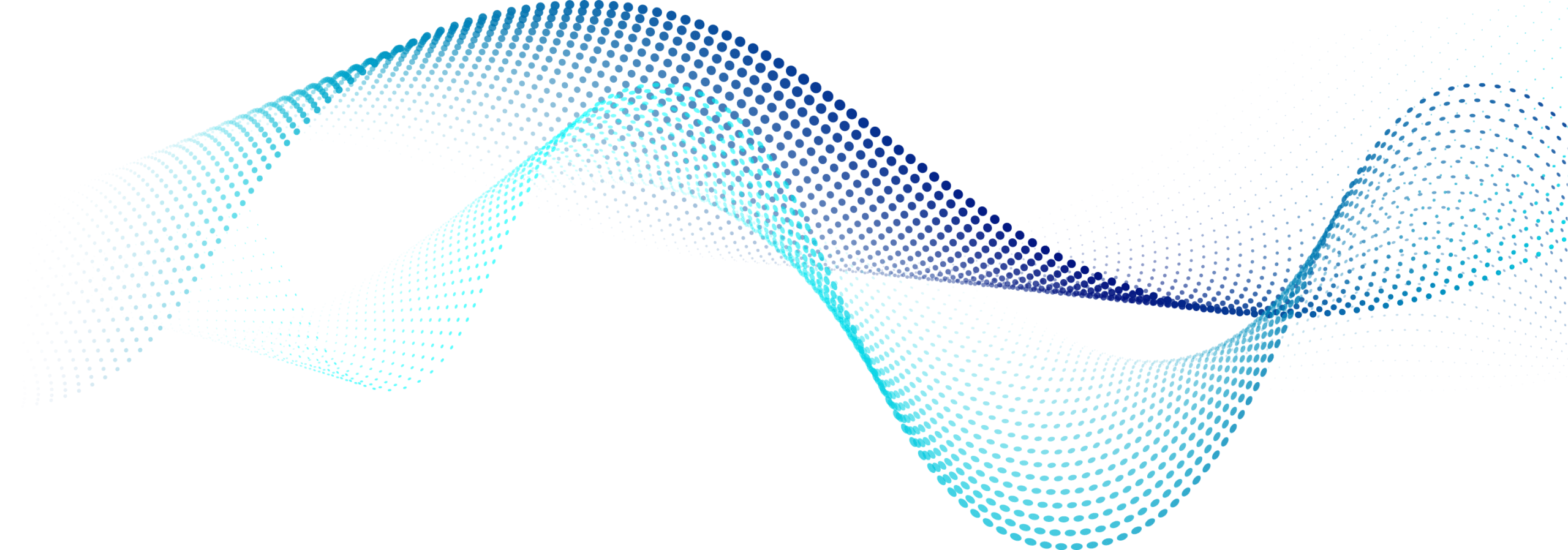
Newsroom
Reltio Newsroom
Press Releases
Media Coverage
Media Coverage
If you are an editor, reporter, or industry analyst who wants to learn more about Reltio or interview one of our executives or other subject matter experts, contact:
Alan Ryan
Allison + Partners
reltio@allisonpr.com
(847) 702-0490