Step up to continuous data quality with modern master data management
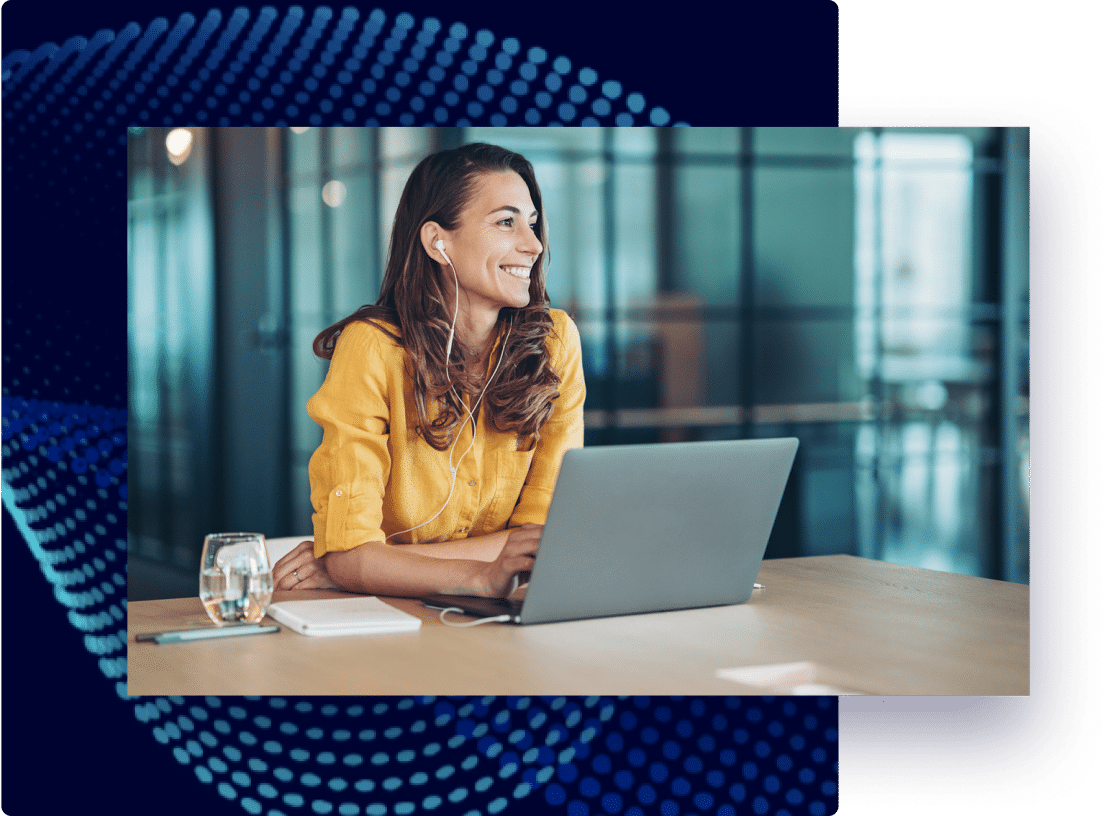
Today’s organizations need high-quality data to thrive. It is at the center of many business transformation initiatives. And as the volume, variety, and velocity of data increases, the quality and availability of data become even more important than ever. As a driver for change, organizations are connecting their core enterprise data and using it to power intelligent systems—from reporting to predictive analytics, from decision support systems to smart processes. So improving the data quality of your data should be one of your top priorities.
Changing data quality demands modern technology solutions
Data is clearly not static. Neither is data quality. Yet data quality processes often focus on quality at a point in time. Traditionally, it starts by evaluating a snapshot of data through a data quality tool once, making minor changes, and then loading the information into a data management solution. After completion, data quality is evaluated infrequently—or more likely never again.
Traditional approaches to data quality do not account for unexpected and undocumented changes to data structure, semantics, and infrastructure that are a result of modern data architectures. Today, the average enterprise has over 500 connected data sources—with larger enterprises having more than 1,000 sources. As the volume and complexity of information continues to grow, it becomes impossible for data stewards to maintain the same caliber of quality. This can result in inaccurate, duplicated, or outdated data to corrupt your golden records and create operational inefficiencies, poor customer experiences, bad decisions, and more—ultimately leading to a negative impact on top-line growth.
Fortunately, we are witnessing the emergence of tools to proactively monitor your data in real-time, provide recommendations, and immediately alert the necessary stakeholders of concerns that need attention. These tools enable the data steward to manage an increasing number of data sources while only having to focus on managing identified problems—not on tracking every source system as it changes over time. This makes it much easier for data stewards to find “needle in the haystack” problems, as they can now use intelligence-based insights to make faster and more informed decisions.
Finding and fixing data quality issues—fast
Data quality tools should drive clarity and fast resolution of data issues. Traditionally, data stewards would need to determine when the issue was first identified, what changed, and where the change came from. Next, they would need to identify who owned the upstream source system and monitor that any communicated issues were resolved in a timely manner—and communicate to downstream stakeholders when the fix was applied. Much of this analysis and communication involves manual human interactions that can often take weeks to complete. Not only is this a bad allocation of time and resources, but throughout the several weeks of updates, bad data would continue to flow into downstream stakeholders systems and create problems. At scale, this process is unmanageable (and costly), but many data stewards today still follow a manual process because they are unaware of alternative solutions.
Modern data quality technology provides proactive ways to identify and resolve upstream data problems with only limited manual effort required from the data steward. Using automation—some of which might include machine learning (ML) to identify and resolve issues—and interactive dashboards to help with observability is increasing in popularity across the entire data pipeline.
Data stewards—the unsung heroes
Data stewards often work behind the scenes. Their work impacts the entire organization, yet most business stakeholders and consumers are unaware of the significant amount of time and energy put into making things “just work.” Using modern data management technologies and practices can enable them to become more efficient and effective while also reducing costs.
The modern data management suite should track the quality of information over time, enabling the business stakeholder and data steward to communicate on a single platform that helps everyone align on a narrative around data quality and the effort invested in tracking and monetizing data. Furthermore, if we are able to track and monitor the business use cases created by downstream stakeholders, we will get much closer to measuring the value of high quality data across the organization.
Take proactive control of your data quality with Reltio
At Reltio, we believe that data should move you forward—not hold you back. Our industry-first, continuous, and automated data quality management helps you take proactive control of your data quality so you can achieve better business outcomes. And it’s an integral part of our Reltio Connected Data Platform—included at no extra cost.
We help you take control of data quality with these key capabilities:
- Continuous data validation so you can automatically identify and correct data issues quickly and easily
- Real-time dashboards that highlight data issues for quick resolution and provide operational insights, trends, and community-driven industry benchmarks
- Upcoming ML-based recommendations to rapidly and accurately identify bad data and remediate it with one click
- Data cleansers so you can cleanse and correct data from all sources as it flows into our platform
- Automated matching including ML capabilities to match and merge profiles from all sources quickly and easily
- Universal ID (UID) to ensure consistent operations across organizations
- Reference data management to standardize data regardless of its source
Modern, continuous data quality management made easy
Proactive, automated, and continuous data quality management practices are now within reach. They enable data stewards to be more productive, while speeding the identification, notification, and resolution of data quality issues. To hear the perspective of Noel Yuhanna from Forrester Research on the latest real-time data quality trends and best practices, and get the details of Advarra’s rapid integration success with around $100K cost savings, I invite you to register for our seminar “The Path to Real-Time, Trusted Data: Emerging Data Trends and Best Practices.“