Unlocking AI/ML Success with Trusted, Unified Data
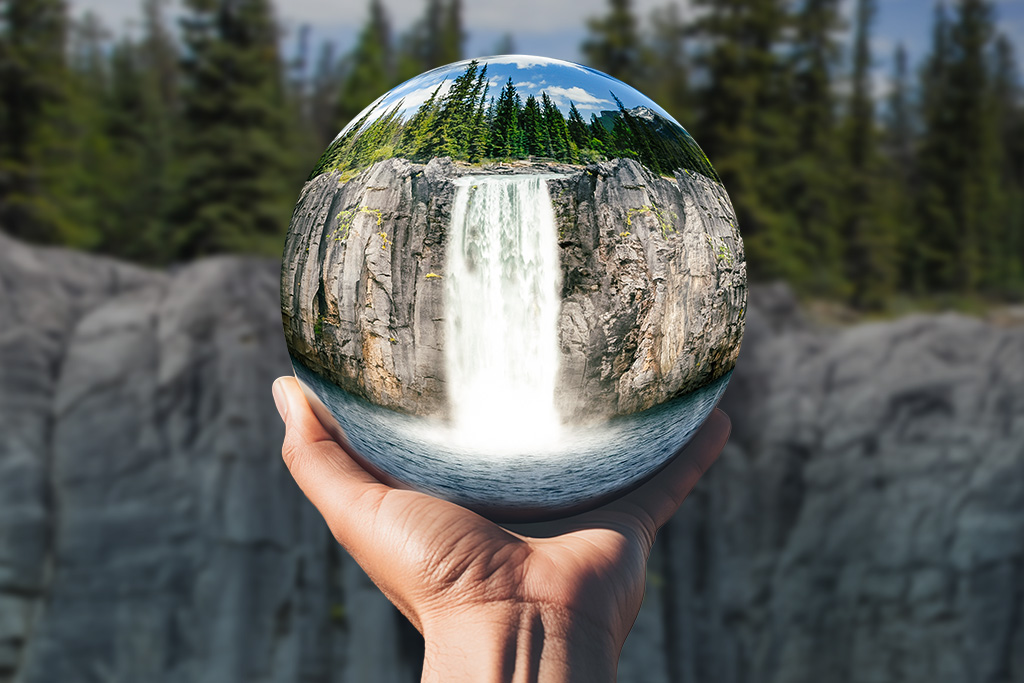
Imagine a skilled chef trying to cook you a gourmet entrée with rotten ingredients or making you soup with polluted water; your dish won’t turn out great. It might even make you sick! Similarly, for businesses using any AI, including machine learning and gen AI, to make decisions or automate processes, data quality is as crucial as the technology itself. Like a meal prepared with subpar ingredients, AI solutions won’t deliver the desired results when fueled with bad data, and those investments won’t pay off.
Consider also this further complication to unlocking AI’s potential: Data scientists spend 50 to 80 percent of their time cleaning and prepping data instead of improving the AI models. And even after all that work, the AI insights are still not as good as they could be. Kind of a letdown, right? It’s like serving an underwhelming meal after all that effort in the kitchen. In this blog post, we highlight how poor data quality hurts enterprise AI initiatives and how to build a foundation on trusted information.
The biggest roadblock to AI/ML success: Bad data
Bad data can undermine enterprise AI/ML initiatives in numerous ways. Poor quality data can lead to inaccurate models, flawed decision-making, and suboptimal automation, ultimately hindering the organization’s ability to achieve its AI/ML goals. From biased or incomplete datasets to inconsistent formatting and errors, bad data can manifest in various forms, each posing unique challenges to the success of AI-driven projects. Here are the top ways poor data quality and governance hurts your AI initiatives:
- Unreliable, inaccurate insights and actions: If your customer data is not complete, up to date, and centered around a trusted unified profile, you may be segmenting that customer inaccurately. Your AI tool might suggest products that are popular among a certain demographic but not necessarily relevant to the specific customer.
- Long data preparation cycles: Without a good data foundation, your data science and data engineering teams will spend unnecessary time and effort preparing the data sets so that the AI tool can use them and feature extraction can be performed effectively and efficiently, as applicable.
- Concerns around AI governance: Weak data governance, whether impacting data privacy, security, traceability, or quality management and monitoring, have compliance consequences that cannot be ignored. The ability to build trust around your AI practice and adopt ethical AI starts with your data practices.
- Delays in launching new capabilities; falling behind competition: The lack of trust in data and the lengthy process to get the data ready for AI initiatives, ultimately delay your ability to advance your business processes with the intelligent, automated skills that your customers demand and likely your competitors have already launched.
Data considerations for AI/ML applications
Considering these data-related barriers and risks, let’s review how to improve your data foundation to enable efficient and ethical AI. Whether for generative AI or predictive analytics, investing in data quality, richness, and governance is the key to “delicious” AI/ML outcomes. Trusted data “ingredients” will strengthen the recommendations and insights you receive from your downstream AI/ML systems. Here are some of the key data considerations when setting up your AI solution:
- Continuous data quality: Consistency, completeness, and data accuracy are essential to training ML models well and then using them in production. You probably have data quality checks in place, but the quality isn’t static—it degrades over time. So if your approach only focuses on point-in-time data quality improvements, it probably isn’t good enough. However, with continuous data quality monitoring, you can ensure that your AI/ML systems always have access to high-quality data.
- Adequate attributes: A dataset with comprehensive, rich attributes can train your ML models better—to identify complex patterns, exhibit better accuracy with edge cases, and reduce bias. Seamless integration with various source systems and third-party data enrichment sources is essential for achieving this capability.
- Data governance: Strong data governance builds trust in ML predictions and helps your organization adhere to privacy regulations.
- Trusted data in milliseconds: Dealing with poor-quality data means spending extra time on manual preprocessing. That could mean minutes, hours, or even days. And that means you can’t support AI/ML operational use cases, which require data to be delivered in milliseconds.
- Flexibility in the data model: Data sources and downstream AI/ML systems are always changing. A flexible data foundation and the ability to handle different data types and formats are important to becoming more responsive to new business needs.
Building a trusted foundation for AI and beyond
Cloud-native, AI-driven data unification and management capabilities simplify and accelerate the path to a trusted data foundation. When data science teams access a true 360-degree view of various entities, such as customers, patients, and products that are properly governed, continuously updated, and quality-validated in milliseconds, their data preparation work is significantly reduced. This means that you can rapidly launch new AI solutions with minimal effort and likely faster than your competition. By designing datasets as reusable data assets, each new project can be launched easier and faster.
At Reltio we focus on enabling our customers with trusted, unified data in real time so they can run their AI and other business initiatives effectively and with agility. We offer data unification in different flavors, powered by our Reltio Connected Data Platform which collects, unifies, harmonizes, and mobilizes multidomain, multisource data in milliseconds. You can access secure, clean, standardized core data in milliseconds with Reltio Multidomain Master Data Management (MDM). If you need more comprehensive profiles with interactions and derived attributes for enterprise-wide use cases, we offer the Reltio Customer 360 Data Product that allows you to work with reusable data assets rather than running ad hoc data projects for customer data. As part of it, Reltio Intelligent Assistant (RIA), our gen AI offering for data exploration, helps you seamlessly access and segment your customer data.
Reltio Entity Resolution and our other main offerings come with groundbreaking Flexible Entity Resolution Networks—-secure, private LLM-powered automation— for rule-free, high-accuracy individual matching. We are excited to harness the power of gen AI and large language models (LLM) to maximize efficiency and value for our customers in their journey to unified, real-time data for AI and other key business initiatives. With prebuilt data pipelines for major cloud data warehouses and data lakes, such as Snowflake and Databricks, we enable clean, unified data in real time for AI and other analytics solutions.
In a new white paper, we reviewed key data considerations for AI solutions and best practices. I invite you to review it to assess your readiness for AI and discover how to hydrate your AI solutions with clean data. If you would like to see our offerings closely, please reach out to talk to our technology experts.