Multi-domain, Cloud-native Master Data Management
To thrive in the hyper-connected economy, you need connected and holistic data in real-time. This is accelerating the revolution of master data management (MDM) and reshaping how organizations collect, unify, manage, and use data.
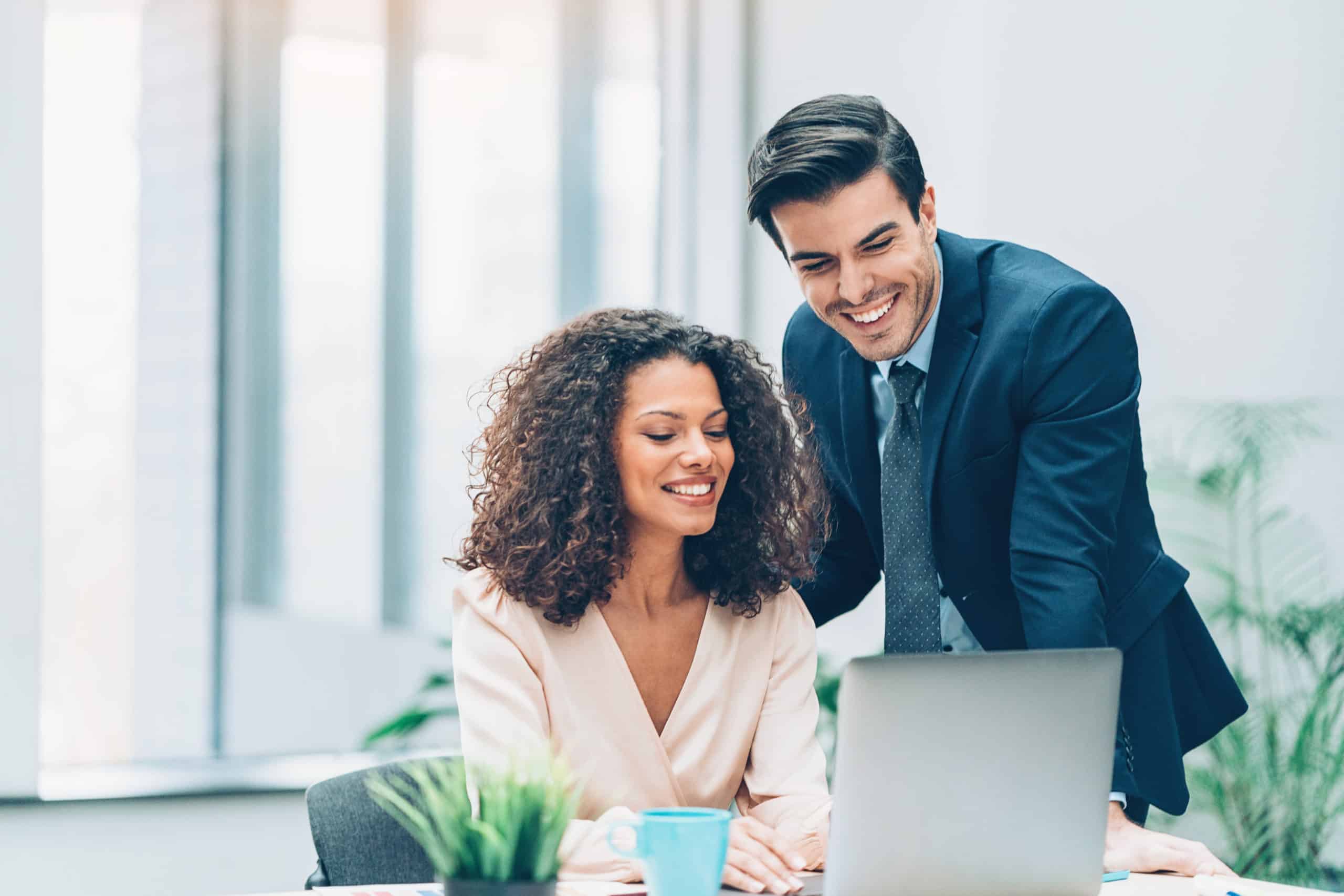
What is Master Data Management?
Gartner defines Master Data Management (MDM) as a “technology-enabled discipline in which business and IT work together to ensure the uniformity, accuracy, stewardship, semantic consistency and accountability of the enterprise’s official shared master data assets. Master data is the consistent and uniform set of identifiers and extended attributes that describes the core entities of the enterprise including customers, prospects, citizens, suppliers, sites, hierarchies, and chart of accounts.”
The digital economy requires organizations to be responsive and have a master data management platform that is connected, scalable, and supports real-time operations. This requires more advanced product data and customer data management strategies and tools, that a more traditional Master Data Management solutions falls short in delivering.
Reltio is the next-generation MDM solution. It offers best in class Master Data Management system capabilities, including Identity Resolution, Data Quality, Dynamic Survivorship for contextual profiles, Universal ID across all your operational applications, Hierarchies, Enterprise Data Management Capabilities and Connected Graph to manage relationships, Progressive Stitching to create richer profiles over time, and Data Governance capabilities.
By making Reltio’s next-generation MDM system the cornerstone of digital transformation data integration initiatives, Innovative Global 2000 companies are leveraging quality data to unlock new opportunities, including support for new business models, hyper-personalized customer engagement, and using artificial intelligence for actionable insights.
Download our checklist to compare the MDM capability of legacy MDM systems vs Reltio’s Modern MDM Solution. Learn how you can improve your Data Management strategy and transform business outcomes.
What differentiates Legacy Master Data Management from Reltio’s Master Data Management Solution?
The differentiation between the legacy master data management systems and Reltio’s cloud-native MDM solution can be boiled down to three areas. First, legacy MDM systems are too slow. Second, they lack the ability to scale effectively. Third, they are too rigid to respond to changing business needs in near real-time.
Reltio’s next-generation MDM solution is a highly secure and compliant cloud-native data management platform. It is available on day one and is easy to configure, so you can go live and gain business value very quickly. All updates are pushed without any disruptions.
Reltio’s MDM solution enables your MDM program with an API first approach, which allows you to quickly onboard new data sources or connect to new consuming systems. You can also add or remove attributes on a fly without downtime.
It enriches 1000s of attributes with:
- Relationships and hierarchies across people, products, and places with connected graph technology.
- Omnichannel Transactions and Interactions including functions, channels brands, and geographies
- Actionable Insights from Data Science and Analytics including preferences, behavior and intent
Consent & Communication Preferences with configurable workflows, reference data, task management, granular audit trails, and support for regulations such as GDPR and CCPA. Learn more about Multi-Domain MDM – Master Data Management below:
On-Demand Webinar: Taking A Customer Experience Approach to Multi-Domain Master Data Management
Cloud-native Master Data Management For All Data, From All Sources, At Big Data Scale
Competitive Advantage | CDP | MDM | DIY | ||
---|---|---|---|---|---|
|
Connected Profiles | ||||
Aggregate all customer data | ![]() |
![]() |
![]() |
![]() |
|
Multi-domain | ![]() |
![]() |
![]() |
![]() |
|
Relationships & hierarchies | ![]() |
![]() |
![]() |
![]() |
|
Agility to change and scale | ![]() |
![]() |
![]() |
![]() |
|
Connected Experiences | |||||
Integrated interactions & transactions | ![]() |
![]() |
![]() |
![]() |
|
Deep profile info for segmentation | ![]() |
![]() |
![]() |
![]() |
|
Compliance & consent management | ![]() |
![]() |
![]() |
![]() |
|
Real-time operation support | ![]() |
![]() |
![]() |
![]() |
|
Actionable Insights | |||||
Rich contextual business views of profiles | ![]() |
![]() |
![]() |
![]() |
|
Insight-ready data for actions | ![]() |
![]() |
![]() |
![]() |
|
Perceived
strength ![]() |








































- Truly Multi-domain MDM Capabilities
Reltio is a next-generation multi-domain cloud MDM platform. It helps you deploy customer, organization, employee, assets, location, and product data masters within the same instance, breaking down data silos. - Comprehensive Profiles
With Reltio you can cleanse, match, merge and unmerge data of any type and domain, including customers, suppliers, employees, locations and products. You can establish a single view, for one source of truth by blending and relating trusted data from a range of internal, external, and third-party sources, references, transactions, interactions and social channels. - Modern Architecture with Connected Graph
Featuring Connected Graph technology, unlike other MDM tools Reltio enables you to store and index an infinite number of attributes and relationships from structured or unstructured data sources including data from disparate systems. In addition, the MDM software and graph technology uncovers many-to-many relationships across people, products, organizations, and locations.
- Machine Learning-based Matching
The Reltio platform combines rule-based and machine learning-based matching. The machine learning-based external match feature allows you to verify and accept match criteria, training the next generation MDM platform to optimize matching and make more accurate predictions as compared to other MDM systems available. - Data Quality Confidence Indicators
Reltio continuously calculates the data quality of all profiles and scores them for users as actionable metrics. This provides a consistent way to quantify data quality to promote reliability, and establishing a trusted data foundation for business analytics. - History & Lineage
Compare and view historical data and the changes with attribute-level granularity. View and control historical customer master data changes across systems, and ensure data quality and integrity with ease.
Reltio named a Leader in Forrester Wave™ 2021 for MDM, and is highlighted as “a one-stop-shop for MDM deployment with advanced capabilities.”
Business Agility. Enterprise Scale.
- Realize quick time to value with only cloud-native MDM, through SaaS deployment and pre-configured data models.
- Boost agility by adapting to changing customer data sources and attributes, without any tedious customizations or downtime.
- Empower business and data users with consumer-grade user interfaces with contextual information.
- Scale to support real-time operations for thousands of users and billions of searches per day with only cloud MDM solution in the market.
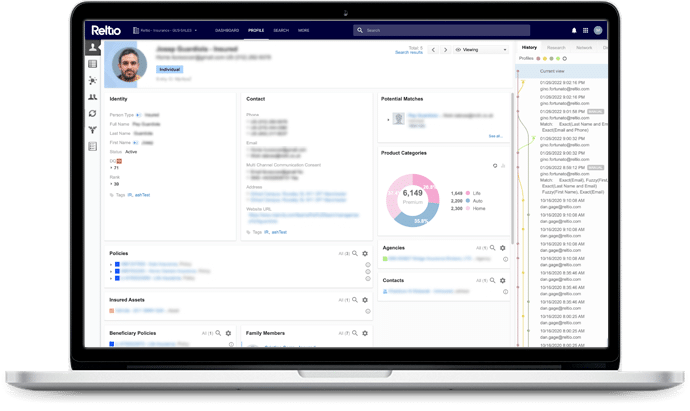